机器学习 / machine learning
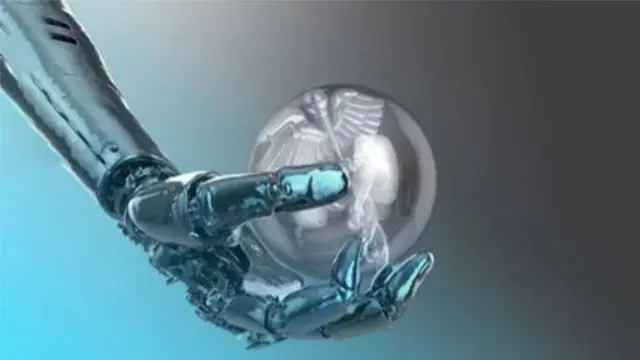
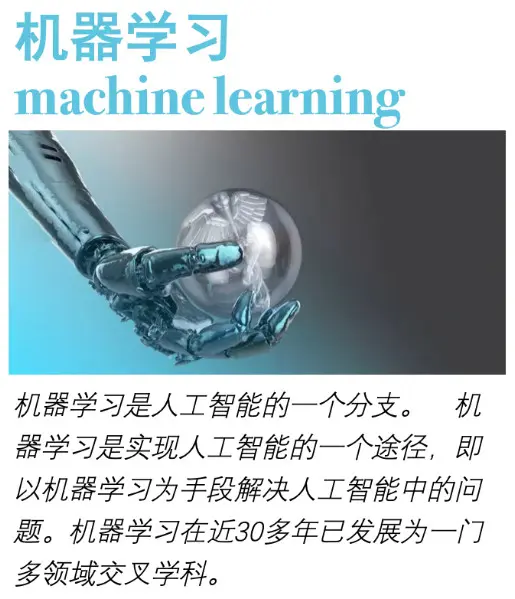
「释义」
机器学习是人工智能的一个分支。
人工智能的研究历史有着一条从以“推理”为重点,到以“知识”为重点,再到以“学习”为重点的自然、清晰的脉络。
机器学习是实现人工智能的一个途径,即以机器学习为手段解决人工智能中的问题。机器学习在近30多年已发展为一门多领域交叉学科。
「应用场景」
过去十年人类在人工智能,即机器学习的各个方面都取得了卓越进展。亚马逊、苹果、Facebook和谷歌等科技巨头利用这种通过数据输入进行预测的技术,极大改进了自身产品。很多初创企业也借此推出新产品和平台,有时甚至可以和大型技术公司相抗衡。
The past decade has brought tremendous advances in an exciting dimension of artificial intelligence—machine learning. This technique for taking data inputs and turning them into predictions has enabled tech giants such as Amazon, apple, Facebook, and Google to dramatically improve their products. It has also spurred start-ups to launch new products and platforms, sometimes even in competition with Big Tech.
位于多伦多的初创企业BenchSci就是如此,该公司致力于缩短药物研发流程。医药公司内部数据库和公开发表的科研论文数量庞大,科学家搜索时无异于大海捞针,该公司的目标是降低信息筛选难度,将精力集中在其中最关键的信息上。为筛选可供临床实验的新药,科学家需要进行大量耗时耗钱的实验。BenchSci公司注意到如果科学家能从已经进行的大量实验中提取更精准的信息,可以减少实验数量,取得更多成功。
Consider BenchSci, a Toronto-based company that seeks to speed the drug development process. It aims to make it easier for scientists to find needles in haystacks—to zero in on the most crucial information embedded in pharma companies’ internal databases and in the vast wealth of published scientific research. To get a new drug candidate into clinical trials, scientists must run costly and time-consuming experiments. BenchSci realized that scientists could conduct fewer of these—and achieve greater success—if they applied better insights from the huge number of experiments that had already been run.
以上文字选自《哈佛商业评论》中文版2020年11月刊《机器学习的制胜之道》
阿杰伊·阿格拉沃尔(Ajay Agrawal)约书亚·甘斯(Joshua Gans)阿维·戈德法布(Avi Goldfarb)丨文
马冰仑 丨编辑