TOPSIS—熵权法的基本原理与基于Python实现代码
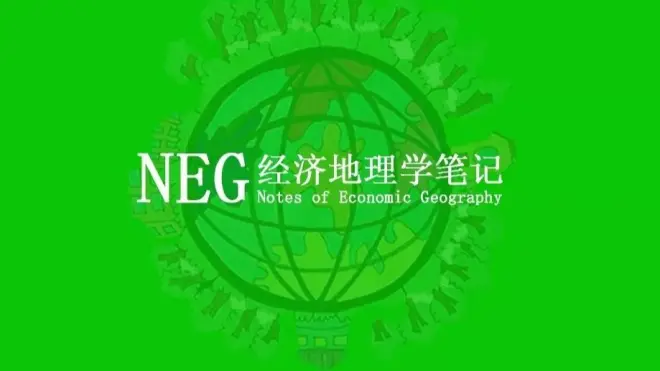
你好,这是必应。根据我的搜索结果,TOPSIS—熵权法综合评价的基本原理是:TOPSIS法(Technique for Order Preference by Similarity to Ideal Solution)可翻译为逼近理想解排序法,是一种常用的综合评价方法,其能充分利用原始数据的信息,其结果能精确地反映各评价方案之间的差距。熵权法是一种客观确定指标权重的方法,可以避免主观因素的影响。TOPSIS与熵权法可以组合使用,通过以下步骤进行综合评价:
指标标准化并得到权重。
得到加权后的规范化矩阵Z。
确定正、负理想解。
计算各样本距离正、负理想解的距离。
计算各评价对象与最优方案的贴近程度。
TOPSIS—熵权法综合评价的公式如下:
标准化矩阵P:
熵值E:
权重W:
加权规范化矩阵Z:
正理想解和负理想解:
距离和:
贴近程度:
代码实现
import numpy as np
def entropy_weight(x):
# 计算每个指标的熵值
m, n = x.shape
e = np.zeros((1, n))
for j in range(n):
p = x[:, j] / x[:, j].sum()
e[0][j] = - (p * np.log(p)).sum()
# 计算每个指标的权重
w = np.zeros((1, n))
for j in range(n):
w[0][j] = (1 - e[0][j]) / ((1 - e).sum())
return w
def topsis(x, w):
# 将x归一化处理
m, n = x.shape
x_norm = np.zeros((m, n))
for j in range(n):
x_norm[:, j] = x[:, j] / np.sqrt((x[:, j]**2).sum())
# 计算加权后的矩阵
x_weighted = np.zeros((m, n))
for j in range(n):
x_weighted[:, j] = w[0][j] * x_norm[:, j]
# 计算最优解和最劣解
max_vec = x_weighted.max(axis=0)
min_vec = x_weighted.min(axis=0)
# 计算每个评价对象与最优解和最劣解的距离
d_plus = np.sqrt(((x_weighted - max_vec)**2).sum(axis=1))
d_minus = np.sqrt(((x_weighted - min_vec)**2).sum(axis=1))
# 计算得分
score = d_minus / (d_minus + d_plus)
return score
# 示例数据
x = np.array([[1,2,3],[4,5,6],[7,8,9]])
# 计算熵权法得到的权重
w = entropy_weight(x)
# 计算TOPSIS得分
score = topsis(x, w)
print(score)
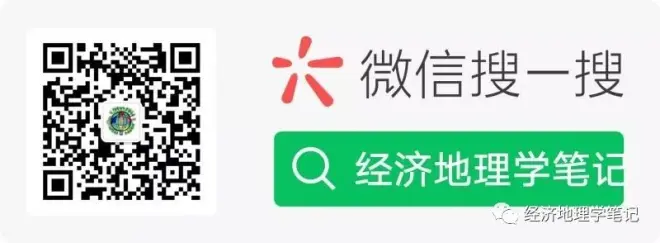
本文使用 文章同步助手 同步