关于IVprobit模型边际效应显著性的一点讨论
Marginal effects significance vs original model effects significance - Statalist
关于IVprobit模型边际效应显著性与原始模型效应显著性对比:statalist
https://www.statalist.org/forums/forum/general-stata-discussion/general/1329201-marginal-effects-significance-vs-original-model-effects-significance

Richard Williams:(贴主)
I often get questions like "This variable has significant effects in the original (logit/probit/heckprobit/whatever) model. But its marginal effect is not significant. Why?" Or vice-versa. I tend to just say that different hypotheses are being tested. But can somebody give a more elegant or complete explanation?
我经常会遇到这样的问题:"这个变量在原始(logit/probit/heckprobit/什么的)模型中效果显著。但其边际效应并不显著。为什么?或者反之亦然。我倾向于只说正在检验不同的假设。但有人能给出更优雅或更完整的解释吗?
Clyde Schechter:
I think this is a multi-faceted problem and that different misunderstandings underlie this question when asked by different people. Among them:
我认为这是一个多方面的问题,不同的人在提出这个问题时会产生不同的误解。其中包括
1. Some are making a fetish out of 0.05. They see a p-value of 0.04 in one place and 0.06 in another where they expect them to be "the same" and they freak out.
1.有些人迷信 0.05。他们看到一个地方的 p 值是 0.04,而另一个地方的 p 值是 0.06,他们希望两者 "一样",于是他们就“抓狂”了。
2.Some have a fundamental misconception of what statistical significance is. They think that statistically significant means "there is an effect" and not statistically significant means "there is no effect." Which again leads them to freak out when they get these apparently contradictory results. For these people, what is needed is re-education about the concept of statistical significance. They need to learn that "statistical significance" is an arbitrary dichotomization of a continuum of degrees of improbability of the outcome under the null hypothesis. I try to get them to think of it differently: a statistically significant result means a combination of the effect in the sample being large enough, and the data being of adequate quantity and quality (low noise) that our estimates of the effect have enough precision that we are highly skeptical of the idea that the true effect size is zero. So our p-values are telling us only indirectly about how big the effect is, and not even actually telling us whether or not it really is zero.
2. 有些人对什么是统计学意义有根本性的误解。他们认为统计意义显著意味着 "有影响",而统计意义不显著意味着 "没有影响"。这再次导致他们在得到这些明显矛盾的结果时抓狂。对于这些人来说,需要的是对统计学意义概念的再教育。他们需要知道,"统计显著性 "是对零假设下结果不可能程度连续体的任意二分法。我试图让他们换个角度思考:统计意义上的结果意味着样本中的效应足够大,而且数据的数量和质量足够好(噪声低),我们对效应的估计有足够的精确度,以至于我们对真实效应大小为零的想法持高度怀疑态度。因此,我们的 p 值只能间接地告诉我们效应有多大,甚至不能告诉我们效应是否真的为零。
I generally prefer to focus them on the confidence intervals, because those are more conducive to thinking about an estimate and the precision of that estimate. Then the p-value can be understood as telling us whether our estimate is both sufficiently far from zero and sufficiently price that it is implausible that we would get such an estimate from a truly zero effect. I also like to point out that in most real-life research situations, the null hypothesis of zero effect is a rather shabby straw man in any case. (As you can tell, I"m not a big fan of p-values.)
一般来说,我更愿意把重点放在置信区间上,因为置信区间更有利于思考估计值和估计值的精确度。那么,P 值就可以理解为告诉我们,我们的估计值是否离零足够远,价格是否足够高,以至于我们无法从真正的零效应中得到这样的估计值。我还想指出,在现实生活中的大多数研究中,零效应的零假设无论如何都是一个相当寒碜的稻草人。(正如你所知道的,我不太喜欢 p 值)。
As an antidote to #1 and #2 I often advise those I mentor not to use the term "statistically significant" in my presence.
The above two are generic and give rise to a lot of misunderstandings and confusion about results from a wide variety of analyses.
作为#1和#2的解药,我经常建议我的导师不要在我面前使用“统计上显著”这个词。
上面两个是通用的,从各种各样的分析结果引起了很多误解和混乱。
More specific to the situations you mention are the following:
更具体到你提到的情况有以下几种:
3. Failure to take into account that the coefficients and odds ratios are different metrics from the marginal effect on probability. The non-linearity of these models then produces "paradoxical" results. In fact, in many situations, if you run -margins, dydx(x) at(x = (numlist spanning a wide range of values)) you will get an interesting mix of large and small, "significant" and "non-significant" marginal effects. Drawing a graph of the logistic curve and pointing out that it has a steep section in the middle and flat sections at the ends probably makes the point better than any number of words and sentences. So a unit increment in x may correspond to a rather large increase in predicted outcome probability if we are starting out in the steep area, and a barely visible increase if we are out at the far ends. Once again, due to the noise in our data and sampling variation, we are estimating these marginal effects with a certain degree of precision, and some, but not all, marginal effects will be large enough that we can bound them away from zero at that degree of precision.
3. 没有考虑到系数和几率比是不同于概率边际效应的指标。这些模型的非线性就会产生 "自相矛盾 "的结果。事实上,在很多情况下,如果运行-margins, dydx(x) at(x = (numlist spanning a wide range of values)),你会得到一个有趣的混合结果,即边际效应有大有小,有 "显著 "的,也有 "不显著 "的。画一张逻辑曲线图,指出它的中间部分比较陡峭,两端比较平坦,可能比任何文字和句子都更能说明问题。因此,如果我们从陡峭区域开始,x 的单位增量可能对应于预测结果概率的大幅增加,而如果我们在远端,则几乎看不到增加。同样,由于数据中的噪声和抽样变化,我们在估算这些边际效应时会有一定的精确度,有些边际效应会大到足以让我们在这种精确度下将其从零束缚开来,但并非所有边际效应都是如此。
Just where we are on the logistic curve isn't always obvious from looking at the regression output or the marginal effects, as it depends on the sample distributions of the predictor variables too. The predicted probability for the sample as a whole, or with all variables set at their means, can be helpful for figuring that out. Once you know where you are on the curve, it is easier to see graphically why a marginal effect might be surprisingly large or small in the face of a particular logistic regression coefficient.
从回归结果或边际效应来看,我们在逻辑曲线上的位置并不总是很明显,因为这也取决于预测变量的样本分布。样本整体的预测概率,或将所有变量设置为均值时的预测概率,可以帮助我们弄清这一点。一旦知道自己在曲线上的位置,就更容易从图形上看出为什么边际效应在特定的逻辑回归系数面前会出奇地大或小。
Stephen Jenkins:
+1 to the post from Clyde. Very nicely put.
My tuppenceworth worth relates to Rich's statement that:I tend to just say that different hypotheses are being tested.
+1克莱德。说得非常好。
我的一点看法与里奇的发言有关,他说:我倾向于只说正在测试不同的假设。
Marginal effects are typically (but not always) non-linear functions of all the estimated parameters and explanatory variables. So even if particular coefficient or OR is "statistically significant", it doesn't guarantee that the marginal effect associated with that coefficient is "statistically significant". For that reason, you can get some of the features described by Clyde in #2.
边际效应通常(但不总是)是所有估计参数和解释变量的非线性函数。因此,即使特定系数或 OR 具有 "统计显著性",也不能保证与该系数相关的边际效应具有 "统计显著性"。因此,你可以得到克莱德在 #2 中描述的一些特征。
Chandra Shah:
Clyde's first point is trivial and that is not the point of the question.The question is not really about why odds ratio is "significant" while the corresponding marginal effect is not. It is really about what does it mean when the respective p-values are very 'far' apart (e.g. the p-value for the odds ratio is .002 an that for the marginal effect is .5). Is this plausible and under what conditions? I accept Stephen's point that the calculation of the marginal effects are typically non-linear functions of all the estimated parameters and explanatory variables. But does this always mean less precision in its calculation? Under what conditions would you get the opposite result? Certainly if you get such large non-trivial differences in the p-values then it is important ask the question about could possibly be the cause.
克莱德的第一点微不足道,这不是问题的重点。实际上,问题并不在于为什么几率比是 "显著的",而相应的边际效应却不显著。真正的问题是,当各自的 p 值相差很 "远 "时(例如,几率比例的 p 值是 0.002,而边际效应的 p 值是 0.5),这意味着什么?这合理吗?我接受斯蒂芬的观点,即边际效应的计算通常是所有估计参数和解释变量的非线性函数。但这是否总是意味着计算精度较低?在什么情况下会得到相反的结果?当然,如果您得到的 p 值差异如此之大,那么就有必要问一问可能的原因是什么。
Stephen Jenkins:
I would agree with Chandra's remark that:
Certainly if you get such large non-trivial differences in the p-values then it is important ask the question about could possibly be the cause.
我同意钱德拉的观点
当然,如果 p 值出现如此大的非微小差异,那么就有必要提出一个问题:原因可能是什么?
However, I doubt that it's possible to set out, in a general way, the conditions under which "large" divergences appear. Won't it be model-specific? Also, it's likely to be related to how non-linear is the function relating the original statistic of interest and the marginal effect of interest. (And remember that there is no single marginal effect in a non-linear model, so "which marginal effect?" is something for Richard as well.) In this respect, Clyde's point 3 is definitely relevant here.
然而,我怀疑是否有可能笼统地列出出现 "大 "分歧的条件。这难道不是因模型而异吗?而且,这很可能与相关原始统计量和相关边际效应之间的非线性函数有关。(请记住,在非线性模型中不存在单一的边际效应,因此 "哪个边际效应?"也是理查德需要考虑的问题)。在这方面,克莱德的第 3 点无疑与此有关。
Richard Williams:
Excellent points, and I especially like how Clyde explains point 3. Different hyp are being tested, And, as Stephen adds, there is no single marginal effect. The value of the marginal effect is contingent on how the values of the other variables in the model are set. You may use atmeans or asobserved and get a single number but there are any number of other ways you could set the values of the other variables.
说得非常好,我尤其喜欢克莱德对第 3 点的解释。正如斯蒂芬所补充的,没有单一的边际效应。边际效应的值取决于模型中其他变量值的设定。您可以使用 atmeans 或 asobserved 得到一个单一的数字,但您也可以通过其他多种方式设置其他变量的值。
Personally, I mostly focus on the significance of coefficients, not the significance of the marginal effect. Or, if I do look at the significance of the marginal effect, it might be over a range of values. So, for example, the marginal effect of race might be very small at low values of age but much greater at higher values of age. e.g. something like
就我个人而言,我主要关注系数的显著性,而不是边际效应的显著性。或者说,如果我真的要看边际效应的显著性,那也可能是在一个数值范围内。例如,在年龄值较低时,种族的边际效应可能非常小,但在年龄值较高时,种族的边际效应就会大得多。
Code:
webuse nhanes2f, clear
svy: logit diabetes i.black i.female age, nolog
margins, dydx(black) at(age=(20 30 40 50 60 70)) vsquish
marginsplot
Chandra Shah:
Quote from Greene:
An empirical conundrum can arise when doing inference about partial effects rather than coefficients. For any particular variable, wk, the preceding theory does not guarantee that both the estimated coefficient, θk and the associated partial effect, δk will both be ‘statistically significant,’ or statistically insignificant. In the event of a conflict, one is left with the uncomfortable problem of simultaneously rejecting and not rejecting the hypothesis that a variable should appear in the model. Opinions differ on how to proceed. Arguably, the inference should be about θk, not δk, since in the latter case, one is testing a hypothesis about a function of all the coefficients, not just the one of interest.
引自格林:
在推断部分效应而非系数时,可能会出现一个经验难题。对于任何特定变量 wk,前面的理论并不能保证估计系数 θk 和相关的部分效应 δk 都 "在统计上显著 "或 "在统计上不显著"。在出现冲突的情况下,我们就会面临一个令人不安的问题,即同时拒绝和不拒绝某个变量应出现在模型中的假设。对于如何处理这个问题,众说纷纭。可以说,推论的对象应该是 θk,而不是 δk,因为在后一种情况下,我们是在检验关于所有系数的函数的假设,而不仅仅是感兴趣的系数。
And I suppose in the case of a bivariate probit with sample selection, the calculation of δk is much more complicated involving parameter estimates and variables from the selection equation as well.
而且我认为,在有样本选择的二元 probit 的情况下,δk 的计算要复杂得多,其中还涉及参数估计和选择方程中的变量。
Does this mean you are more likely to encounter such conundrums when you have a more complex model like a bivariate probit with sample selection than a simple probit?
Is this anything to do with the consistency of the estimator for the variance-covariance matrix?Or the delta method used in the calculation?
这是否意味着,与简单的 probit 模型相比,在使用带有样本选择的双变量 probit 这样更复杂的模型时,更容易遇到这样的难题?
这是否与方差-协方差矩阵估计器的一致性有关?还是计算中使用的 delta 方法?
Stephen Jenkins:
Please provide an exact and full bibliographic citation for the quotation from Greene.
I would suggest that the issues are primarily to do with how non-linear the transformations are that relate the original parameter(s) to the partial/marginal effects. (And not the issues raised in your last 2 questions.)
请提供引自 Greene 的确切、完整的参考文献。
我认为,这些问题主要与将原始参数与部分/边际效应联系起来的变换的非线性程度有关。(而不是你在最后两个问题中提出的问题)。
Marcos Almeida:
I kindly ask you to provide quotes, well, under quotation marks (as shown above), as recommended in the FAQ. By reading #7, it turns out difficult to many of us to spotlight who said who, I mean, message and unmarked quotation, unfortunately, got entwined.
我恳请您按照常见问题的建议,在引号下(如上图所示)提供引文。通过阅读 #7,我们中的很多人都很难发现是谁说了谁的话,我的意思是,不幸的是,信息和未加标记的引文纠缠在了一起。
Chandra Shah:(回应引文)
page 12: http://archive.nyu.edu/bitstream/2451/26036/2/7-7.pdf
Also, see:
Dowd, BE, Greene, WH & Norton, EC 2014, 'Computation of Standard Errors', Health Services Research, vol. 49, pp. 731-750.
where the authors discuss the issue of consistency of the estimator of variance- covariance matrix and the complexity involved in calculating the standard error of the a marginal effect in a multiple equation model (e.g. bivariate probit with sample selection). If full information maximum likelihood is used then the estimator is consistent.
Sorry I can't figure out how to do the quotes like in the above posts!
第 12 页:http://archive.nyu.edu/bitstream/2451/26036/2/7-7.pdf
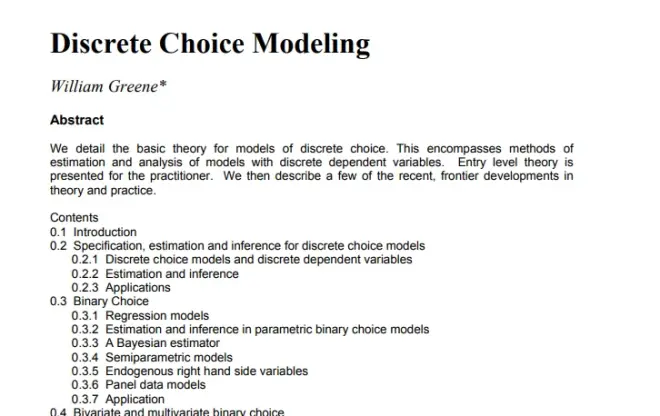
另见,
Dowd, BE, Greene, WH & Norton, EC 2014, 'Computation of Standard Errors', Health Services Research, vol. 49, pp.
作者在书中讨论了方差-协方差矩阵估计值的一致性问题,以及计算多方程模型(如带有样本选择的双变量 probit)中边际效应标准误差的复杂性。如果使用全信息最大似然法,那么估计值是一致的。
抱歉,我不知道如何像上面的帖子那样使用引号!
Stephen Jenkins:
Chandra: please read the FAQ from top to bottom. (Hit the black bar at the top of the page.) Your post #11 suggests you have mastered how to do the "quote" inserts, but also read about using CODE delimiters. Also read how to use the Advanced editor and its functionality (accessed by clicking on the underlined upper-case A in the editing box for composing messages). With that you can insert hyperlinked URLs
Chandra: 请从上到下阅读常见问题(点击页面顶部的黑条)。你的帖子 #11 表明你已经掌握了如何插入 "引用",但也请阅读有关使用 CODE 分隔符的内容。还请阅读如何使用高级编辑器及其功能(点击编辑框中的下划线大写 A 即可进入)。有了它,你可以插入超链接 URL
For Stata's capabilities for estimating marginal effects for a "bivariate probit with sample selection", see help heckprobit_postestimation. Also read the associated manual entry for information about methods and formulae. The "complexity" you refer to exists of course, but StataCorp have done a lot of work for you.
关于 Stata 估算 "带样本选择的双变量 probit "边际效应的功能,请参阅 help heckprobit_postestimation。还可以阅读相关的手册条目,了解有关方法和公式的信息。您所说的 "复杂性 " (δk 的计算要复杂得多,其中还涉及参数估计和选择方程中的变量) 当然存在,但 StataCorp 已经为您做了大量工作。
Chandra Shah:
Thanks Stephen, just found the Advanced editor!
谢谢你,斯蒂芬,我刚找到高级编辑器!
I have used Stata capabilities you refer to for estimating my bivariate model with sample selection. Both the outcome and selection equations are important for the research questions I am investigating. Furthermore I have replicate weights, plausible values and missing data to deal with. For each plausible value I have three multiple imputed data sets, making 30 multiple imputed data. Incidentally the two variables for which the p-values of the coeff. and the marginal effect are radically 'far' apart are both continuous.
And thanks everybody for your contribution to this thread.
我使用了您提到的 Stata 功能来估计带有样本选择的双变量模型。结果方程和选择方程对我正在研究的问题都很重要。此外,我还需要处理重复权重、可信值和缺失数据。对于每个似然值,我都有三个多重估算数据集,即 30 个多重估算数据。顺便提一下,系数和边际效应的 p 值相差很远的两个变量都是连续变量。
感谢大家对本主题的贡献。
Richard Williams:
That kind of complexity makes my head hurt! I might try skipping a few of the bells and whistles (e.g. do it without the imputation, or without the replicate weights) and see if that changes anything. I wouldn't expect it to, but a mistake somewhere along the way (either by you or by Stata) might affect the results.
这种复杂性让我头疼!我可能会试着跳过一些繁琐的程序(例如,不进行估算,或不使用重复权重),看看是否会有什么变化。我不指望会有什么变化,但过程中的某个错误(无论是你还是 Stata)可能会影响结果。
Sebastian Kripfganz:
From a technical perspective, Stephen's answer is already sufficient. Coefficients and marginal effects are different quantities, and the latter are often non-linear combinations of the former. Typically, one would expect the p-values to be similar but there is no reason why they have to be close or even identical.
从技术角度看,斯蒂芬的回答已经足够了(将原始参数与部分/边际效应联系起来的变换的非线性程度)。系数和边际效应是不同的量,后者往往是前者的非线性组合。通常情况下,我们会希望 p 值相似,但没有理由说它们必须接近甚至相同。
With regard to the discussion whether to look at coefficients or p-values, we should ask: Why are we interested in the respective test result? The coefficients themselves in non-linear models typically do not have a good interpretation when we want to examine the effect of a certain covariate on our outcome variable. When interpreting the results, we would typically look at the marginal effects. Yet, testing for statistical significance of the coefficient estimates can be relevant when we think about the model specification, whether to include or exclude a certain covariate.
关于是看系数还是看 p 值的讨论,我们应该问:为什么我们对各自的检验结果感兴趣?当我们想研究某个协变量对结果变量的影响时,非线性模型中的系数本身通常没有很好的解释。在解释结果时,我们通常会关注边际效应。然而,当我们考虑模型的规格时,检验系数估计值的统计显著性可能与我们的考虑相关,即是否包含或排除某个协变量。
A variable could be statistically relevant in the sense that its coefficient estimate is statistically significant but at the same time its marginal effect might be statistically insignificant. The latter does not mean that this variable does not matter because it also affects the marginal effects of all the other covariates. In other words, including it still improves the fit of the model.
一个变量在统计意义上可能是相关的,即其系数估计值在统计意义上是显著的,但同时其边际效应在统计意义上可能是不显著的。后者并不意味着该变量不重要,因为它也会影响所有其他协变量的边际效应。换句话说,加入该变量仍能改善模型的拟合效果。
Chandra Shah:
Thanks for the latest comments.
As you rightly point out Richard, the results are in the same ball park without multiple imputation and replicate weights. But I only know this after having estimated the models both ways! There were 10% missing values in aggregate across all variables. As Paul Allison noted, one should impute missing values whenever possible. Also the use of replicate weights and plausible values is what the OECD recommend for the analysis of the PIAAC data that I am using.
感谢您的最新评论。
正如您正确指出的那样,理查德(这种复杂性让我头痛!),在没有多重估算和重复权重的情况下,结果是相同的。但我是在对两种方法的模型都进行了估计之后才知道这一点的!所有变量的缺失值合计为 10%。正如保罗-埃里森(Paul Allison)所指出的,只要有可能,就应该对缺失值进行估算。此外,经合组织也建议在分析我正在使用的 PIAAC 数据时使用重复权重和可信值。
Elise Sobrie:(also我的疑问)
Dear all
After reading a couple of times your posts, I understand what you are saying. However, it is not clear to me whether to follow the p-values of the margins or those from the coefficients in the Original model. I know different hypotheses etc. are beign tested, but just one clear answer which to follow?
Thank you in advance!
亲爱的各位
读了几遍你们的帖子后,我明白了你们的意思。但是,我不清楚是应该遵循边际的 p 值,还是遵循原始模型中系数的 p 值。我知道要对不同的假设等进行检验,但只想得到一个明确的答案,到底应该遵循哪个?
在此先表示感谢!
Richard Williams:(回应Elise)
As I already stated, my preference is to use the p-values from the original coefficients. I think that is also what is more common. Many analyses do not even present adjusted predictions or marginal effects, but you just about always have the coefficients.
But I am not the ultimate authority on such things, so you should decide what is best for you given what you want to test.
我已经说过,我更倾向于使用原始系数的 p 值。我认为这也是更常见的做法。很多分析甚至没有提出调整后的预测值或边际效应,但你几乎总是有系数。
但我不是这方面的终极权威,所以您应该根据自己想要测试的内容来决定哪种方法最适合您。
Evelyn Mare:
I have encountered a similar problem. My logit models suggests a p value of <.05 and my logit model a p value >.15.
Originally posted by Richard Williams View Post
As I already stated, my preference is to use the p-values from the original coefficients. I think that is also what is more common
我也遇到了类似的问题。我的 logit 模型显示 p 值小于 0.05,而我的 logit 模型显示 p 值大于 0.15。“理查德:我已经说过,我倾向于使用原始系数的 p 值。我认为这也是更常见的做法”
I would lean towards presenting the AMEs, because they are easier to interpret, yet I am wrangling with the insignificance.
我倾向于提出 AMEs,因为它们更容易解释,但我也在纠结其微不足道之处。
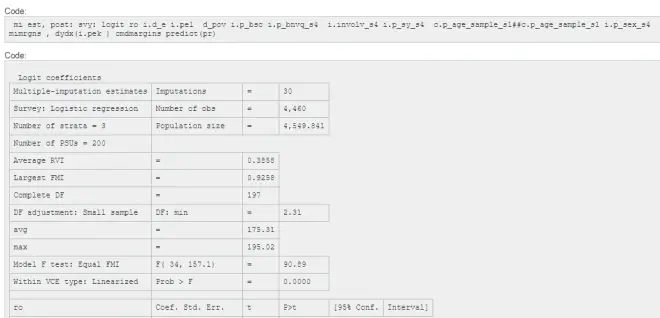
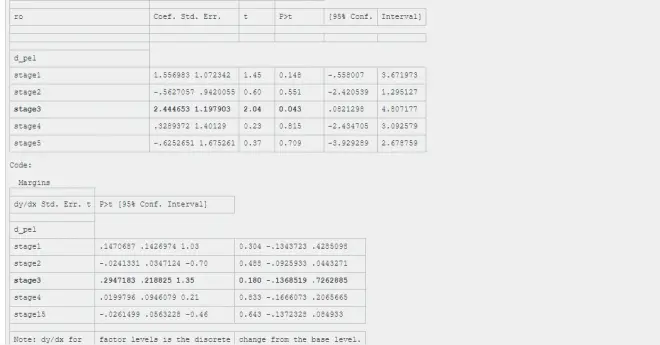
Edmondo Ricci:
Dear Clyde,
Thank you for your post. Since tests on marginal effect and coefficients are different tests, I can see statistical significance can be different.
But would it be even possible that coefficients are positive and marginal effect is negative?
I managed to make a dataset where I get following results.
亲爱的克莱德
感谢您的来信。由于边际效应检验和系数检验是不同的检验方法,我知道统计意义可能不同。
但是,系数是正值而边际效应是负值,这可能吗?
我设法制作了一个数据集,得到了以下结果。
1. reg => positive
2. ivreg => positive
3. probit coefficient => positive
4. probit marginal effect => positive
5. ivprobit coefficient => positive
6. ivprobit marginal effect => negative
1. 回归 => 正
2. ivreg => 正
3. probit 系数 => 正
4. probit 边际效应 => 正
5. ivprobit 系数 => 正
6. ivprobit 边际效应 => 负
This is strange in so many levels. #5 and #6 having different sign is strange. #2 and #6 having different sign is strange.
And if this is possible, in which situation would it occur?
And if this is possible, shouldn't there be somewhere in the range of x such that ivprobit marginal effect is positive?
And how should I interpret this? Is X causing increase in Y or decrease in Y? All others are positive & significant. Only marginal effects from ivprobit is negative (and sometimes significant)
这在很多方面都很奇怪。#5 号和 6 号的符号不同很奇怪。#2 和 #6 的符号不同也很奇怪。
如果有可能,在什么情况下会出现这种情况?
如果有可能,难道不应该在 x 范围内的某个地方出现 ivprobit 边际效应为正的情况吗?
我该如何解释?X 是导致 Y 增加还是 Y 减少?所有其他变量都是正的、显著的。只有 ivprobit 的边际效应是负的(有时是显著的)。
Clyde Schechter:(回复Edmondo)
I'm unable to respond to your specific question because I do not use instrumental variables in my work and I have only a very limited understanding of how they work.
I can say that with linear models, the marginal effect is equal to the coefficient. With non-linear models, the marginal effect must be conditioned on particular values of the predictor variables (or averaged over the distribution of the predictor variables) and can vary considerably, whereas regression coefficients are unconditional. So there is no necessary type of agreement between a regression coefficient and all of the infinitely many marginal effects associated with that variable.
我无法回答您的具体问题,因为我的工作中不使用工具变量,而且我对工具变量的工作原理了解非常有限。
我可以说,在线性模型中,边际效应等于系数。对于非线性模型,边际效应必须以预测变量的特定值(或预测变量分布的平均值)为条件,而且可能会有很大的变化,而回归系数是无条件的。因此,回归系数与与该变量相关的无限多的边际效应之间并不存在必然的一致。
Teresa Schützeichel:
Following this thread, I am still confused as to why the significance levels of the ivprobit original coefficients and those of its average marginal effects should not be identical. I ran an ivprobit which returned positive and significant coefficients (varying p-values for the different specifications), however the corresponding average marginal effects after running margins, dydx(*) predict (pr) were all positive and insignificant (p>0.1 for all specifications).
The other thing is the command 'margins, dydx (*) predict (pr)' returns the average marginal effects of the instrumental variable as well, ideally this should not happen..any idea why this is the case?
Thanks in advance.
跟随着这个主题,我仍然感到困惑的是,为什么 ivprobit 原始系数的显著性水平与其平均边际效应的显著性水平不一致。我运行了 ivprobit,得到的系数为正且显著(不同规格的 p 值不同),但运行 margins, dydx(*) predict (pr) 后得到的相应平均边际效应均为正且不显著(所有规格的 p 均大于 0.1)。
另外,"margins, dydx (*) predict (pr) "命令也会返回工具变量的平均边际效应,理想情况下不应该出现这种情况。
在此先表示感谢。
Richard Williams:(回复Teresa)
Teresa, welcome to Statalist.
i suggest reading the Statalist FAQ, esp. point 12 about asking Qs effectively. Showing exactly what you typed and how Stata responded can make it easier to see what you are talking about.
Also, while I might add a link to old threads, I like to start a new thread rather than add on to a long older one. If something has 20+ posts I usually don’t want to go to the trouble of getting up to date on what has already been talked about.
i personally do not get too surprised about difference in significance levels between coefficients and marginal effects. Marginal effects can be computed in many ways, e.g. atmeans, asobserved, or at values chosen by the user. These different ways might produce different significance levels. I usually just focus on the significance of the coefficients.
特雷莎,欢迎来到 Statalist。
我建议您阅读 Statalist 常见问题解答,尤其是关于有效提问的第 12 点。准确显示您输入的内容以及 Stata 是如何回应的,可以让人更容易理解您在说什么。
另外,虽然我可能会添加旧主题的链接,但我喜欢启动一个新主题,而不是添加到一个很长的旧主题中。如果一个主题有 20 多个帖子,我通常就不想再费力去了解已经讨论过的内容。
我个人对系数和边际效应之间显著性水平的差异并不感到太惊讶。边际效应的计算方法有很多种,如按平均值、按观察值或按用户选择的值。这些不同的方法可能会产生不同的显著性水平。我通常只关注系数的显著性。(Williams最后的结论)