【TED】如何建立一个能够获得最佳决策的公司
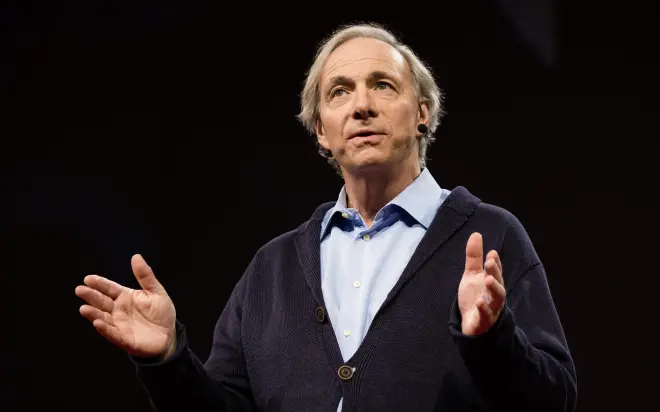
中英文稿
不管你是否喜欢, 绝对透明和利用算法的 决策时代正在快速到来, 并且将改变你的生活。 因为现在把算法嵌入到计算机中 十分容易, 它能收集你所有的 私自保留的信息, 明白哪些是你需要的, 然后引导计算机与你互动, 这比大部分人跟你 互动的效果好多了。 这听上去似乎有点吓人。 不过我这么做已经很久了, 发现其实很管用。 我的目标是做有意义的工作, 与同事建立有意义的工作关系, 我知道,如果没有 绝对透明和算法决策的支持, 这一切都不会发生。 我想告诉你们为什么会这样, 并展示它是如何运作的。
接下来向你们展示的 也许有点不同寻常。 当我还是小孩子时, 我憎恶死记硬背。 不喜欢按部就班, 也不擅长墨守成规。 我喜欢用自己的方式来理解事物。 我12岁的时候, 讨厌上学,但爱上了市场交易。 我当时是个小球童, 服务一包球得5美元。 我把自己的球童收入投入了股市。 因为那个时候股市很火爆。 我买的第一个公司股票是 一家叫做东北航空的公司。 东北航空是当时我所知道 唯一的单股价格低于 5 美元的。 (笑) 我计划买更多的股票, 如果上涨,就可以赚更多的钱。 这个策略听上去挺傻的吧? 不过我赚了3倍的钱, 因为当时运气不错。 那家公司快破产了, 最后被其他公司收购, 我就翻了3倍。 从此就一发不可收拾。 我在想: “这个游戏真简单。” 后来, 我才明白这个游戏一点也不简单。 要成为一个成功的投资人, 要和市场对赌, 还得成功。 要做到这一点很难。 之所以难, 是因为股票价格是由市场决定的。 不过要成为一个 成功的企业家, 你必须与市场对赌并且赢得胜利。
我是个企业家,也是一个投资家, 扮演这种角色的代价就是 获得了很多惨痛的教训。 我的确得到了很多教训, 随后, 我对于犯错的态度转变了。 我开始意识到它们是谜团。 如果我能解开这些谜团, 就会获得巨大的回报。 这个谜团就是: 如何防止我在将来不犯大错? 而要获得回报的原则就是 记录并回忆它们, 这对将来的决策很有帮助。 因为我清楚地做了详细的记录, 最终—— 我发现了—— 我能将这些方法嵌入算法中。 再把算法嵌入计算机中, 计算机就能协助我做决定; 同时,我们也能制定出最后的决策。 和我自己做的决策对比之后, 我发现这些决策要好很多。 因为计算机做决策更快, 能处理更多信息, 几乎不带有任何情绪地 处理更多决策。 于是它彻底提高了 我的决策正确率。
在创建了Bridgewater 8年后, 我犯下了人生中最大的错误, 经历了最大的一次失败。 那是1970年代末, 我当时34岁, 计算出美国银行 出借了太多资金给新兴国家, 而不是那些有能力还款的国家。 这会导致自大萧条后 最大的债务危机。 随后,就会出现经济危机, 股市走熊。 这个观点当时是有争议的, 人们认为我的想法太疯狂。 但是在1982年8月, 墨西哥违约, 其他一系列国家跟进, 的确出现了自大萧条后 最严重的债务危机。 因为我早先的预测, 我被国会要求出席 “华尔街之周”的听证会, 当时备受关注。 我裁剪了一小短现场录像, 大家可以看到我。 (录像)主席先生,米切尔先生, 很荣幸出席今天的会议, 检查我们的经济哪里出了问题。 经济增长已经停滞—— 在失败的边缘摇摇欲坠。 Martin Zweig: 最近有一篇文章提到了你, 你说过:“我对此深信不疑, 我知道市场是如何运作的。” Ray Dalio: 我能毫不犹豫的说, 如果你们在公司和整个世界范围内 观察流动性基础, 流动性大幅度减少, 你不可能回到一个滞胀的时代。“ 现在回过头来看, 我想的是:“多么自负的傻瓜!” (笑) 我太骄傲了,而且大错特错。 我的意思是,尽管债务危机发生了, 股市和经济却都在上涨,而非下降, 我损失了自己和客户的大量资金, 不得不关闭整个公司, 遣散所有的员工。 这是一个延伸的大家庭, 我的心都要碎了。 我本人也损失惨重, 不得不向我父亲借了4000美元 来支付家庭的日常开支。
这是我人生经历中 最惨痛的一次经历。。。 同时也成为我人生经历中 最珍贵的一次经历, 因为它改变了我对决策的态度。 不再去想,“我是正确的”, 我现在开始询问自己 “你如何才知道自己是正确的?” 为了平衡我的鲁莽, 我学会了什么是谦卑。 我需要找到最聪明的人来反驳我, 以此来理解他们的观点, 或者来测试我的观点。 我称之为“精英对话”。 换个说法是, 不利用自己的权利来带领其他人, 也不是每个人都能 平等说出自己观点的民主氛围, 而是用精英对话的方式, 来得到最好的点子。 为了实现这一点, 我意识到公司需要完全的信任, 完全的透明, 我指的绝对诚实和绝对透明, 是指人们能表达出自己的真实想法, 能看得更加全面。 我们会记录下所有的对话, 让每个人都能看清一切, 如果我们不这么做, 就不能实现真正的精英管理。 为了达到这个目的, 我们需要让人们自由表达。
这里有个例子, 这是一封Jim Haskel 写给我的电子邮件—— Jim 是我的员工—— 公司中任何人都可以这样做。 “Ray,针对你今天在会上的表现, 只能给你评个D-, 你根本没有做好准备, 从来没有哪次会议 组织得这么混乱。“ 是不是很棒? (笑) 的确很棒。 因为首先,我需要这样的反馈。 我需要像这样的反馈。 如果我不支持Jim或者其他人 自由表达自己的观点, 我们的关系就会变得不同了。 如果我不让每个人都能看见, 这就不是精英对话。 我们这样做了25年, 保持绝对透明, 凭着这样的原则, 避免了重大错误, 于是我们把这个原则植入了算法中。
算法向我们证明了—— 我们可以跟随算法做决策, 同时也运用我们自己的思考。 这就是我们如何经营投资公司, 以及如何管理员工。 为了让你们更好的理解我的意思, 我想邀请大家参加一个会议, 看看我们的工具“网络收集者” 是如何帮助我们完成目标的。 在美国大选一周后, 我们的调查团队开了个会, 讨论特朗普当选 对美国经济意味着什么。 很自然的,人们对此有很多不同观点, 我们如何进行这次讨论呢? “网络收集器”收集观点 其中列举了几十个属性, 只要思考其他人的想法 就很容易交换评价。 属性提供从1-10的评价系统。 比如,会议开始时, 调查者Jen给我的评分是3—— 换句话说,很糟—— (笑) 在开放和自信的 平衡方面做得不好。
随着会议继续进行, Jen也对其他人也会做出评价, 屋里其他人有各自不同的观点。 这很正常。 不同人总有不同观点, 怎么分辨谁对谁错呢? 只关注一下人们对我的评价。 一些人认为我做的很好, 另外一些人认为很糟。 依据不同的观点, 我们能探索数字背后的原因。 这是Jen和Larry说的。 每人都有机会表达想法, 包括他们的评判式思考, 无论他们在公司里的地位如何。 Jen,24岁,刚从学校毕业, 她能告诉我,他们的CEO, 在解决问题方面很糟。 这个工具帮助人们自由表达, 把观点和个人分离开, 从更高层次看问题。 当Jen和其他人交换观点 并纵览整个屏幕, 他们的观念就会发生转变。 他们看出自己代表的 仅仅是其中的一部分观点, 自然会开始自问, “我怎么知道我的观点是正确的呢?” 观点的转变就像只从一个维度 看多维空间。
谈话从争论各种观点,转变成了 找到客观标准来决定 哪个观点最好。 在“网络收集器”的背后, 计算机正在观察 所有这些人在想什么, 并与他们如何思考相关联。 基于这些谈话反馈, 从所有会议中抽取数据, 对人们的特点和他们的想法 做出数据点图。 这个过程由算法引导, 了解人们的特点可以 帮助他们更好的与工作进行匹配。 例如, 创造性思想家不太靠谱, 也许可以匹配一个靠谱 但是无创造性的人。 知道其他人的想法, 也让我们能够决定 应该赋予他们怎样的责任, 并基于人们的优势 来权衡我们的决策。 我们称之为他们的“可依赖度”。
这里有个我们投票的例子, 大部分人同意一个方案—— 但当我们根据各自优势来权衡时, 答案就不同了。 这个过程让我们做决定的时候 不再基于民主, 不基于专制, 仅基于考虑人们可依赖度的算法。 没错,我们就是这样做的。 (笑) 因为这消除了 人性中最大的悲剧, 就是人类的自大、 天真,自以为是的 持有错误的观点, 并执行它, 而不放在压力环境中进行测试, 就会产生悲剧。 我们相信这样做会提升自己, 开始从别人眼中看问题, 用集体方式的看待问题。 如果运用良好,集体决策比个人决策 要好很多。 这就是我们成功背后的秘密武器。
正因为如此,对比其他对冲基金公司, 我们可以为客户赚更多钱。 26年中有23年都是如此。 那么绝对信任和绝对透明的 问题是什么呢? 人们认为从感情上很难接受。 有批评家说这是 严酷工作环境的模式。 神经科学家告诉我, 这与大脑如何进行预先假设有关。 一部分大脑知道我们会犯错, 直面缺点会让后我们变得更好。 这是前额皮层做的事情。 大脑的另外一部分 会将这些当成抨击的观点。 这是在大脑杏仁核区域发生的。 换句话说,有2个人在你体内, 一个极富情绪, 另一个很理智, 他们彼此常常互不相容, 时常对抗你。 我们的经验表明, 我们可以赢得这场战争, 以团队的方式。 通常需要18个月 去发现大部分人喜欢的方式, 以完全透明, 而非隐蔽的环境中 进行公司改造。 没有政治,没有野蛮—— 也就是没有任何暗箱操作—— 人们可以畅所欲言的表达。 这样很好。 给我们更有效的工作, 以及更有效的人际关系。 但这并不适用于任何人。 我们发现有25%-30%的人 不适合这样做。
顺便提一句, 当我说绝对透明, 不是指对任何事情都绝对透明。 你不需要告诉别人 他们秃顶越来越严重, 或者他们的孩子很难看。 我的意思是—— (笑) 重要的事情。 所以—— (笑) 当你离开这间屋子, 我希望你在与他人交流时 能仔细观察自己, 想象如果你能真正理解他人的思想, 真正理解他人的特质, 想象他们能真正理解你的思想, 理解你的特质。 这样一来一定会 帮你理清很多事情, 使得你们在一起合作更加高效。 我想这样也会促进你们的关系。 现在想象,你有一些算法 帮助你收集信息, 甚至帮助你做出优选决策。 这样绝对透明的时代马上就要到来, 影响你的生活。 依我的拙见, 前途是光明的。 我希望这个方法对你有帮助, 就如同我们所收获的一样。 十分感谢。 (鼓掌)
Whether you like it or not, radical transparency and algorithmic decision-making is coming at you fast, and it's going to change your life. That's because it's now easy to take algorithms and embed them into computers and gather all that data that you're leaving on yourself all over the place, and know what you're like, and then direct the computers to interact with you in ways that are better than most people can.
Well, that might sound scary. I've been doing this for a long time and I have found it to be wonderful. My objective has been to have meaningful work and meaningful relationships with the people I work with, and I've learned that I couldn't have that unless I had that radical transparency and that algorithmic decision-making. I want to show you why that is, I want to show you how it works. And I warn you that some of the things that I'm going to show you probably are a little bit shocking.
Since I was a kid, I've had a terrible rote memory. And I didn't like following instructions, I was no good at following instructions. But I loved to figure out how things worked for myself. When I was 12, I hated school but I fell in love with trading the markets. I caddied at the time, earned about five dollars a bag. And I took my caddying money, and I put it in the stock market. And that was just because the stock market was hot at the time. And the first company I bought was a company by the name of Northeast Airlines. Northeast Airlines was the only company I heard of that was selling for less than five dollars a share.
(Laughter)
And I figured I could buy more shares, and if it went up, I'd make more money. So, it was a dumb strategy, right? But I tripled my money, and I tripled my money because I got lucky. The company was about to go bankrupt, but some other company acquired it, and I tripled my money. And I was hooked. And I thought, "This game is easy." With time, I learned this game is anything but easy.
In order to be an effective investor, one has to bet against the consensus and be right. And it's not easy to bet against the consensus and be right. One has to bet against the consensus and be right because the consensus is built into the price. And in order to be an entrepreneur, a successful entrepreneur, one has to bet against the consensus and be right. I had to be an entrepreneur and an investor -- and what goes along with that is making a lot of painful mistakes. So I made a lot of painful mistakes, and with time, my attitude about those mistakes began to change. I began to think of them as puzzles. That if I could solve the puzzles, they would give me gems. And the puzzles were: What would I do differently in the future so I wouldn't make that painful mistake? And the gems were principles that I would then write down so I would remember them that would help me in the future. And because I wrote them down so clearly, I could then -- eventually discovered -- I could then embed them into algorithms. And those algorithms would be embedded in computers, and the computers would make decisions along with me; and so in parallel, we would make these decisions. And I could see how those decisions then compared with my own decisions, and I could see that those decisions were a lot better. And that was because the computer could make decisions much faster, it could process a lot more information and it can process decisions much more -- less emotionally. So it radically improved my decision-making.
Eight years after I started Bridgewater, I had my greatest failure, my greatest mistake. It was late 1970s, I was 34 years old, and I had calculated that American banks had lent much more money to emerging countries than those countries were going to be able to pay back and that we would have the greatest debt crisis since the Great Depression. And with it, an economic crisis and a big bear market in stocks. It was a controversial view at the time. People thought it was kind of a crazy point of view. But in August 1982, Mexico defaulted on its debt, and a number of other countries followed. And we had the greatest debt crisis since the Great Depression. And because I had anticipated that, I was asked to testify to Congress and appear on "Wall Street Week," which was the show of the time. Just to give you a flavor of that, I've got a clip here, and you'll see me in there.
(Video) Mr. Chairman, Mr. Mitchell, it's a great pleasure and a great honor to be able to appear before you in examination with what is going wrong with our economy. The economy is now flat -- teetering on the brink of failure.
Martin Zweig: You were recently quoted in an article. You said, "I can say this with absolute certainty because I know how markets work."
Ray Dalio: I can say with absolute certainty that if you look at the liquidity base in the corporations and the world as a whole, that there's such reduced level of liquidity that you can't return to an era of stagflation."
I look at that now, I think, "What an arrogant jerk!"
(Laughter)
I was so arrogant, and I was so wrong. I mean, while the debt crisis happened, the stock market and the economy went up rather than going down, and I lost so much money for myself and for my clients that I had to shut down my operation pretty much, I had to let almost everybody go. And these were like extended family, I was heartbroken. And I had lost so much money that I had to borrow 4,000 dollars from my dad to help to pay my family bills.
It was one of the most painful experiences of my life ... but it turned out to be one of the greatest experiences of my life because it changed my attitude about decision-making. Rather than thinking, "I'm right," I started to ask myself, "How do I know I'm right?" I gained a humility that I needed in order to balance my audacity. I wanted to find the smartest people who would disagree with me to try to understand their perspective or to have them stress test my perspective. I wanted to make an idea meritocracy. In other words, not an autocracy in which I would lead and others would follow and not a democracy in which everybody's points of view were equally valued, but I wanted to have an idea meritocracy in which the best ideas would win out. And in order to do that, I realized that we would need radical truthfulness and radical transparency.
What I mean by radical truthfulness and radical transparency is people needed to say what they really believed and to see everything. And we literally tape almost all conversations and let everybody see everything, because if we didn't do that, we couldn't really have an idea meritocracy. In order to have an idea meritocracy, we have let people speak and say what they want. Just to give you an example, this is an email from Jim Haskel -- somebody who works for me -- and this was available to everybody in the company. "Ray, you deserve a 'D-' for your performance today in the meeting ... you did not prepare at all well because there is no way you could have been that disorganized." Isn't that great?
(Laughter)
That's great. It's great because, first of all, I needed feedback like that. I need feedback like that. And it's great because if I don't let Jim, and people like Jim, to express their points of view, our relationship wouldn't be the same. And if I didn't make that public for everybody to see, we wouldn't have an idea meritocracy.
So for that last 25 years that's how we've been operating. We've been operating with this radical transparency and then collecting these principles, largely from making mistakes, and then embedding those principles into algorithms. And then those algorithms provide -- we're following the algorithms in parallel with our thinking. That has been how we've run the investment business, and it's how we also deal with the people management.
In order to give you a glimmer into what this looks like, I'd like to take you into a meeting and introduce you to a tool of ours called the "Dot Collector" that helps us do this. A week after the US election, our research team held a meeting to discuss what a Trump presidency would mean for the US economy. Naturally, people had different opinions on the matter and how we were approaching the discussion. The "Dot Collector" collects these views. It has a list of a few dozen attributes, so whenever somebody thinks something about another person's thinking, it's easy for them to convey their assessment; they simply note the attribute and provide a rating from one to 10. For example, as the meeting began, a researcher named Jen rated me a three -- in other words, badly --
(Laughter)
for not showing a good balance of open-mindedness and assertiveness. As the meeting transpired, Jen's assessments of people added up like this. Others in the room have different opinions. That's normal. Different people are always going to have different opinions. And who knows who's right? Let's look at just what people thought about how I was doing. Some people thought I did well, others, poorly. With each of these views, we can explore the thinking behind the numbers. Here's what Jen and Larry said. Note that everyone gets to express their thinking, including their critical thinking, regardless of their position in the company. Jen, who's 24 years old and right out of college, can tell me, the CEO, that I'm approaching things terribly.
This tool helps people both express their opinions and then separate themselves from their opinions to see things from a higher level. When Jen and others shift their attentions from inputting their own opinions to looking down on the whole screen, their perspective changes. They see their own opinions as just one of many and naturally start asking themselves, "How do I know my opinion is right?" That shift in perspective is like going from seeing in one dimension to seeing in multiple dimensions. And it shifts the conversation from arguing over our opinions to figuring out objective criteria for determining which opinions are best.
Behind the "Dot Collector" is a computer that is watching. It watches what all these people are thinking and it correlates that with how they think. And it communicates advice back to each of them based on that. Then it draws the data from all the meetings to create a pointilist painting of what people are like and how they think. And it does that guided by algorithms. Knowing what people are like helps to match them better with their jobs. For example, a creative thinker who is unreliable might be matched up with someone who's reliable but not creative. Knowing what people are like also allows us to decide what responsibilities to give them and to weigh our decisions based on people's merits. We call it their believability. Here's an example of a vote that we took where the majority of people felt one way ... but when we weighed the views based on people's merits, the answer was completely different. This process allows us to make decisions not based on democracy, not based on autocracy, but based on algorithms that take people's believability into consideration.
Yup, we really do this.
(Laughter)
We do it because it eliminates what I believe to be one of the greatest tragedies of mankind, and that is people arrogantly,******** holding opinions in their minds that are wrong, and acting on them, and not putting them out there to stress test them. And that's a tragedy. And we do it because it elevates ourselves above our own opinions so that we start to see things through everybody's eyes, and we see things collectively. Collective decision-making is so much better than individual decision-making if it's done well. It's been the secret sauce behind our success. It's why we've made more money for our clients than any other hedge fund in existence and made money 23 out of the last 26 years.
So what's the problem with being radically truthful and radically transparent with each other? People say it's emotionally difficult. Critics say it's a formula for a brutal work environment. Neuroscientists tell me it has to do with how are brains are prewired. There's a part of our brain that would like to know our mistakes and like to look at our weaknesses so we could do better. I'm told that that's the prefrontal cortex. And then there's a part of our brain which views all of this as attacks. I'm told that that's the amygdala. In other words, there are two you's inside you: there's an emotional you and there's an intellectual you, and often they're at odds, and often they work against you. It's been our experience that we can win this battle. We win it as a group. It takes about 18 months typically to find that most people prefer operating this way, with this radical transparency than to be operating in a more opaque environment. There's not politics, there's not the brutality of -- you know, all of that hidden, behind-the-scenes -- there's an idea meritocracy where people can speak up. And that's been great. It's given us more effective work, and it's given us more effective relationships. But it's not for everybody. We found something like 25 or 30 percent of the population it's just not for. And by the way, when I say radical transparency, I'm not saying transparency about everything. I mean, you don't have to tell somebody that their bald spot is growing or their baby's ugly. So, I'm just talking about --
(Laughter)
talking about the important things. So --
(Laughter)
So when you leave this room, I'd like you to observe yourself in conversations with others. Imagine if you knew what they were really thinking, and imagine if you knew what they were really like ... and imagine if they knew what you were really thinking and what were really like. It would certainly clear things up a lot and make your operations together more effective. I think it will improve your relationships. Now imagine that you can have algorithms that will help you gather all of that information and even help you make decisions in an idea-meritocratic way. This sort of radical transparency is coming at you and it is going to affect your life. And in my opinion, it's going to be wonderful. So I hope it is as wonderful for you as it is for me.
Thank you very much.
(Applause)