李宏毅对抗生成网络(GAN)国语教程(2018)
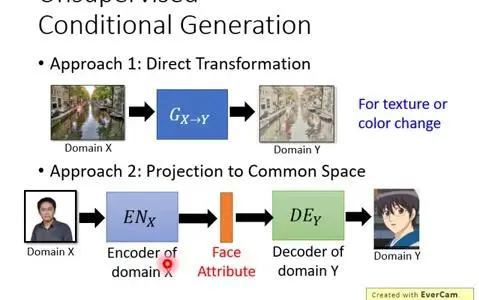
Introduction P1 - 02:26
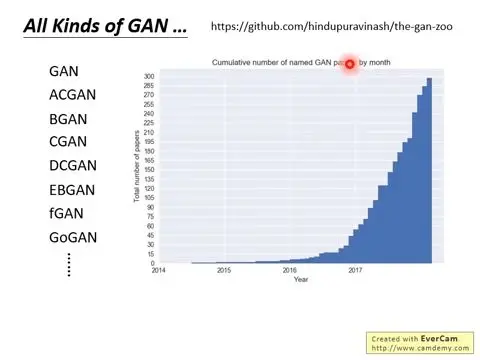
收录了各种有名有姓的gan
Introduction P1 - 04:14
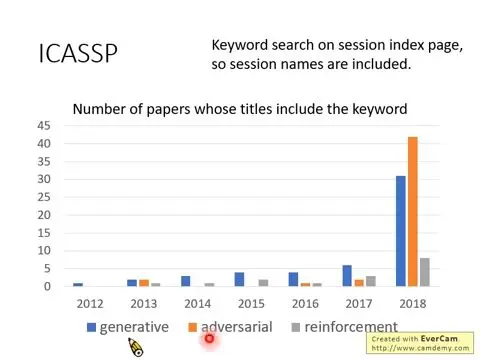
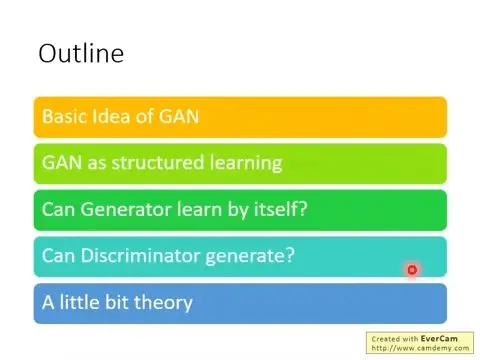
s生成东西:图片、写诗
Introduction P1 - 06:05
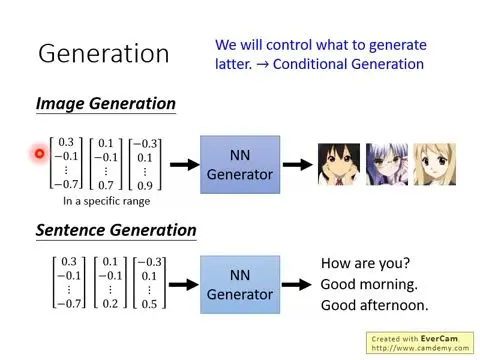
输入文字、产生图片or输入图片、产生另一张图片
s输入一个向量,影像生成:G的输出就是一个高维向量
Introduction P1 - 08:06
Introduction P1 - 09:04
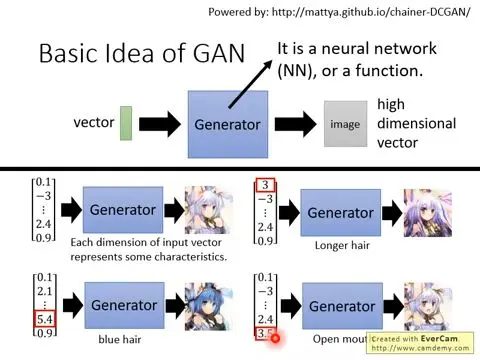
s输出的数字越大,生成的图片越真实
Introduction P1 - 10:42
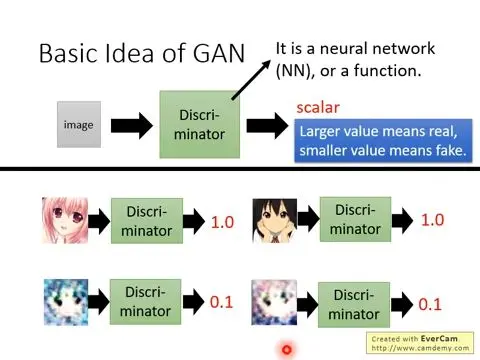
Introduction P1 - 12:47
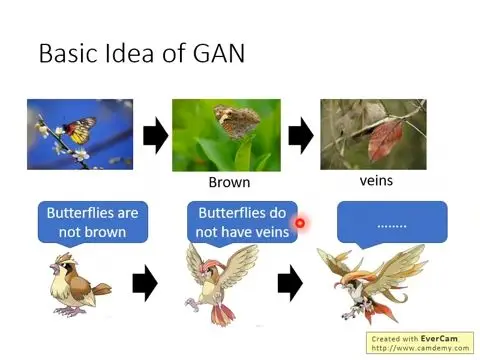
Introduction P1 - 14:57
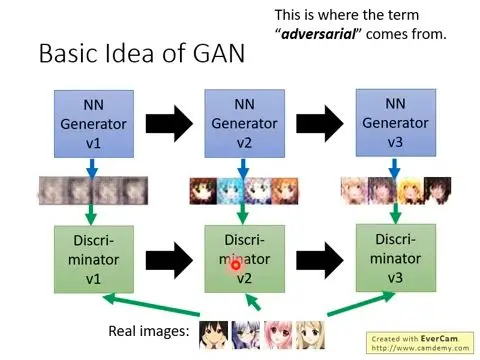
Introduction P1 - 18:15
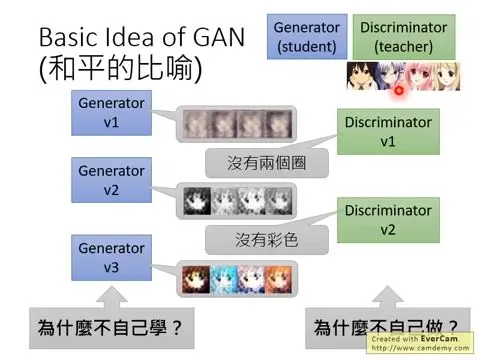
Introduction P1 - 21:49
如果图片是真实的,就给高分;如果是G生成的,就给低分
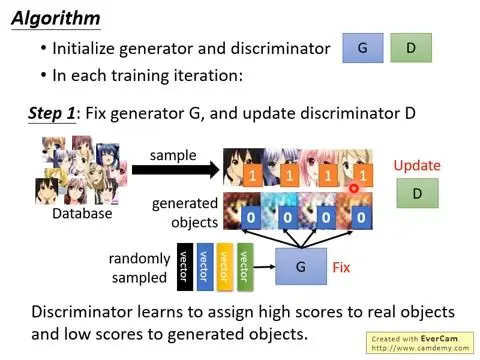
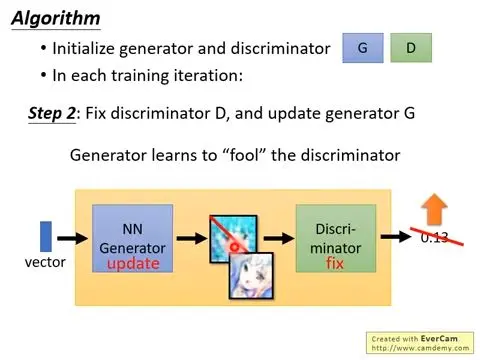
Introduction P1 - 27:01
Introduction P1 - 28:27
前半部分训练D
learning rate怎么调:Adam等进阶的来设置learning rate
最后斯塔d更新几次?是一个需要调的参数,由你自己决定
后半部分训练G
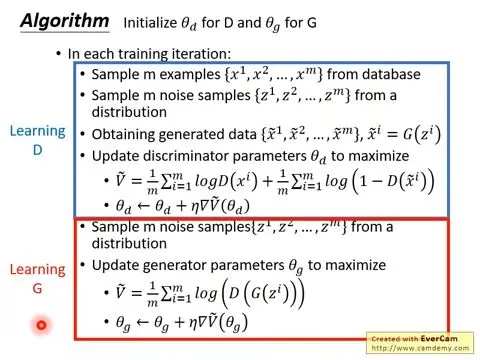
Introduction P1 - 35:23
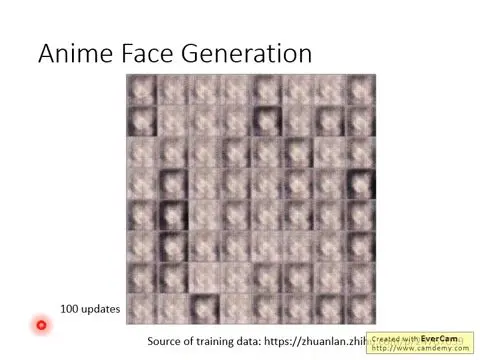
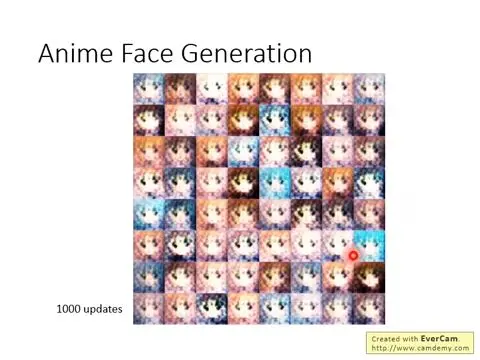
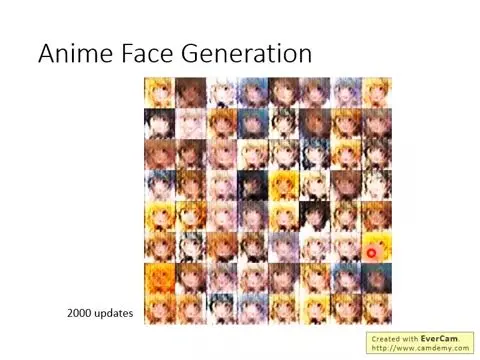
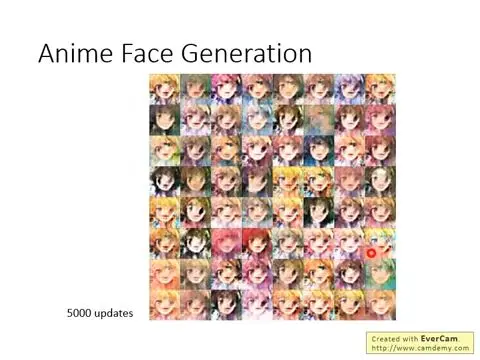
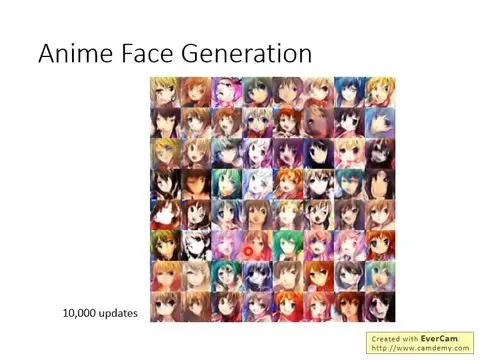
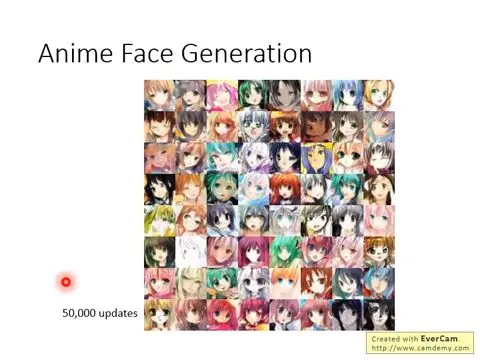
Introduction P1 - 38:16
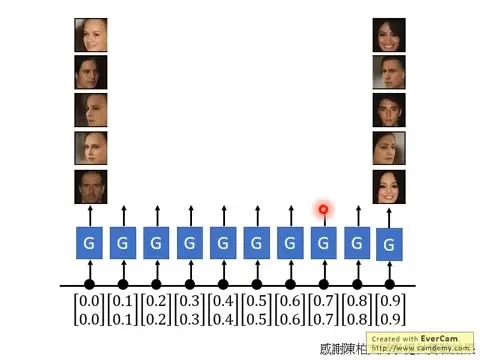
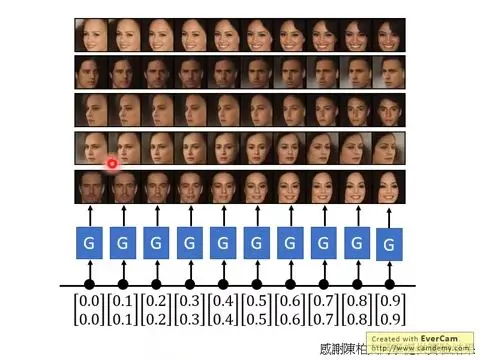
Introduction P1 - 39:54
GAN可以视为structure learning
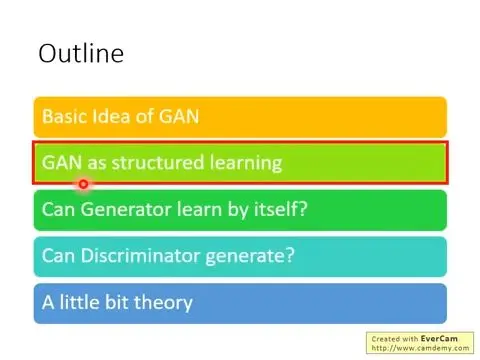
什么是structure learning
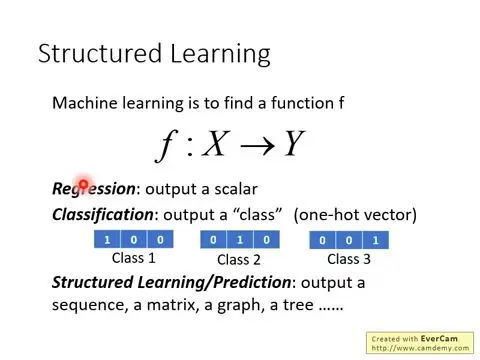
structure learning举例
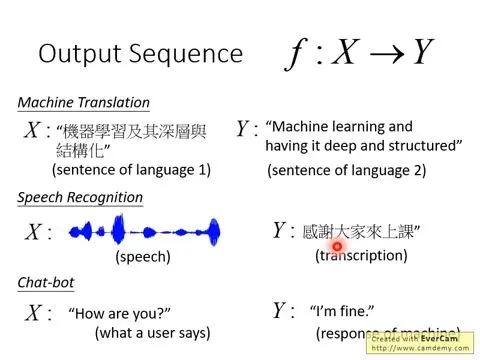
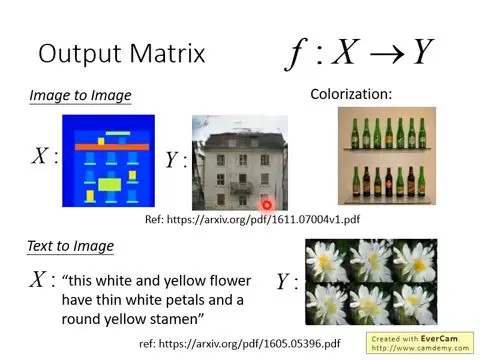
Introduction P1 - 42:08
structure learning是输出的答案可能是training时一次也没见到过的,可以视为一种创造
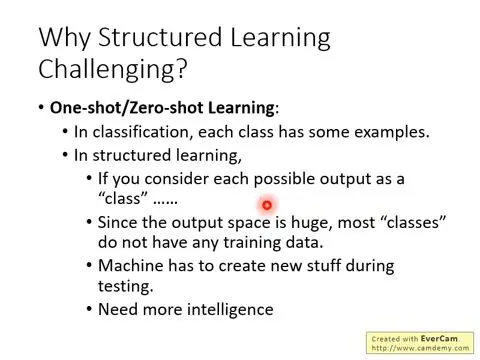
解决structure learning问题必须有规划的概念,大局观
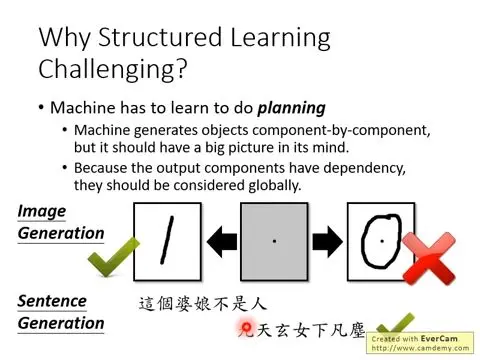
c传统的structure learning两套方法
Introduction P1 - 47:02
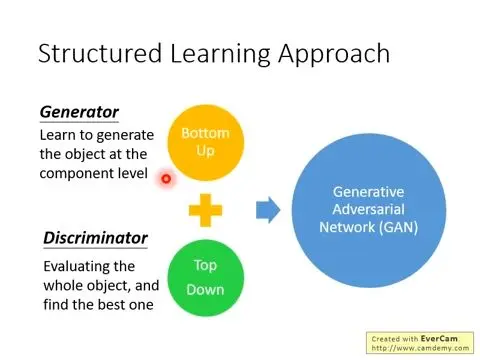
Introduction P1 - 48:05
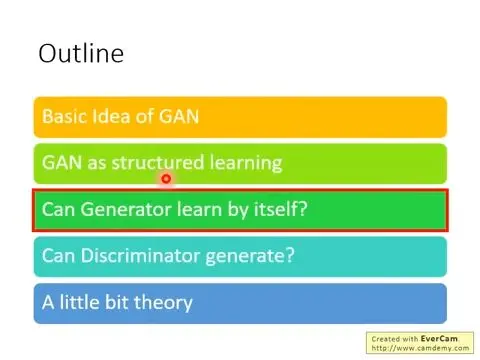
给每一个图片assign一个vector
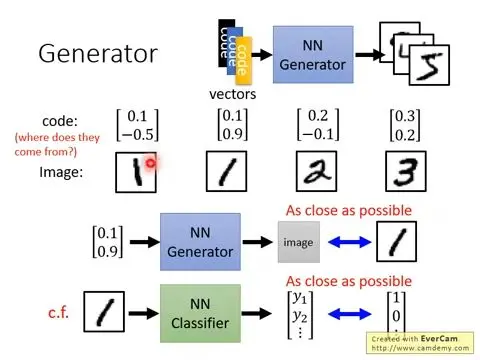
希望:input的vector和output的东西的特征有关系,怎么实现?learn一个encoder,给一个图片,它把图片的特征用向量表示
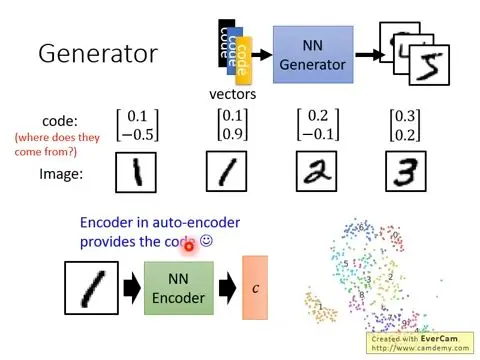
Introduction P1 - 51:22
希望input和output越接近越好
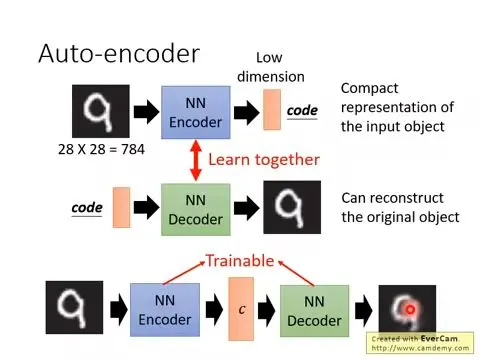
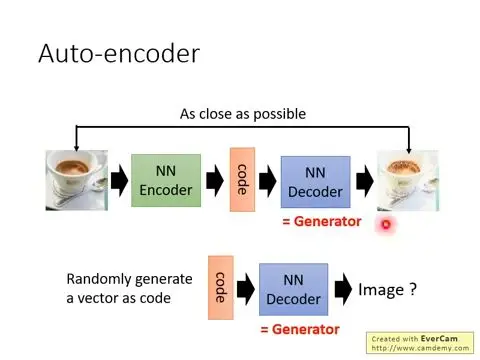
Introduction P1 - 53:01
数字产生器
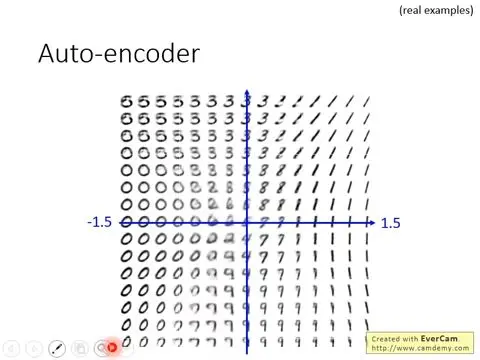
Introduction P1 - 54:01
training-data里的image有限
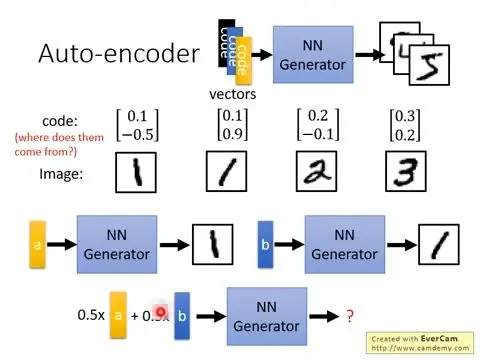
VAE,不仅会产生一个code,还会产生每一个dimension的variance
Introduction P1 - 54:54
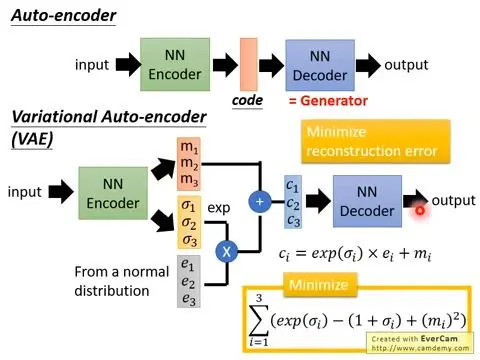
就算输入的vector是训练时从没见过的,output的东西仍然是合理的
Introduction P1 - 56:30
Encoder 的 Dimension 是多少?
Introduction P1 - 58:10
Introduction P1 - 59:43
auto-encoder的training少了什么东西
选择在什么地方妥协
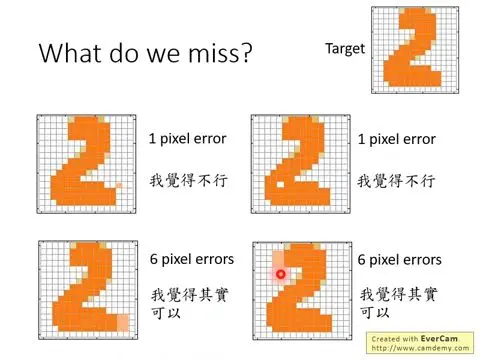
Introduction P1 - 01:03:34
structure learning里面component和component之间的关系很重要
单纯用一个G困难的地方
Introduction P1 - 01:07:50
可以
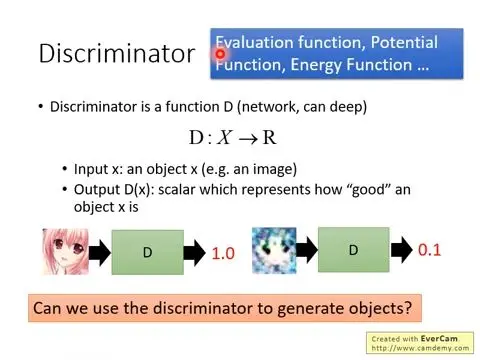
Introduction P1 - 01:09:08
D的优势
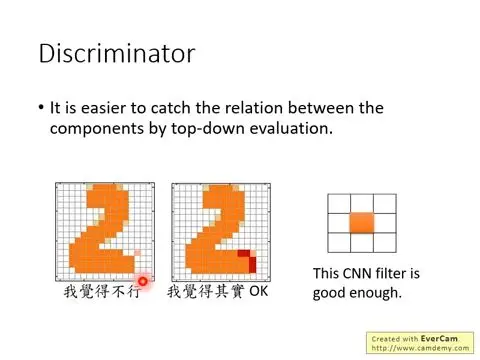
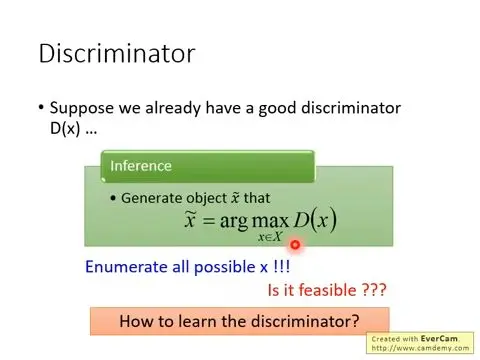
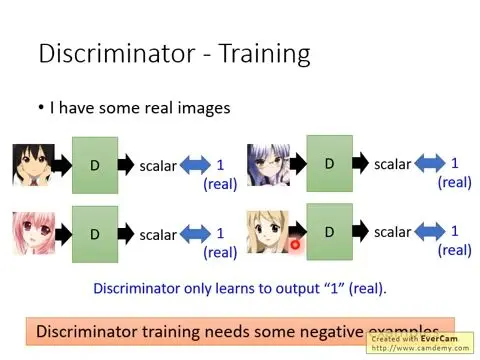
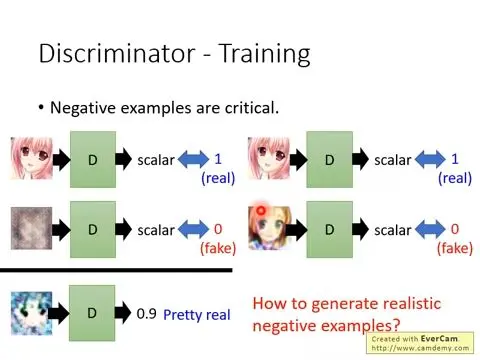
Introduction P1 - 01:15:27
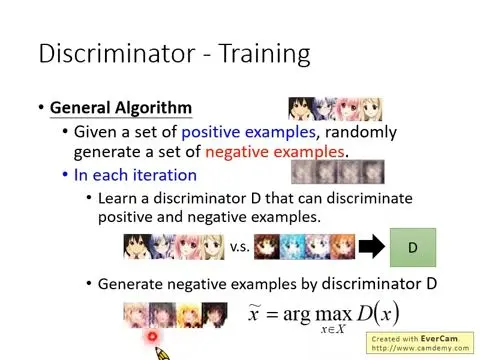
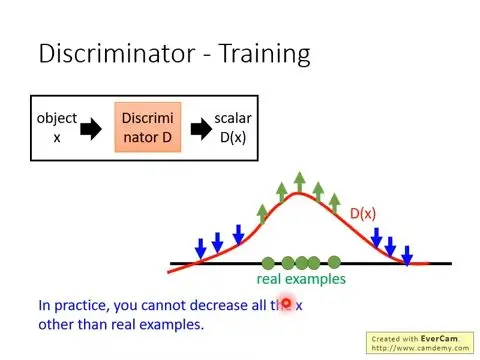
Introduction P1 - 01:18:12
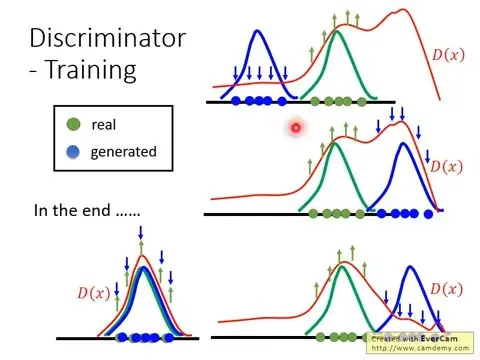
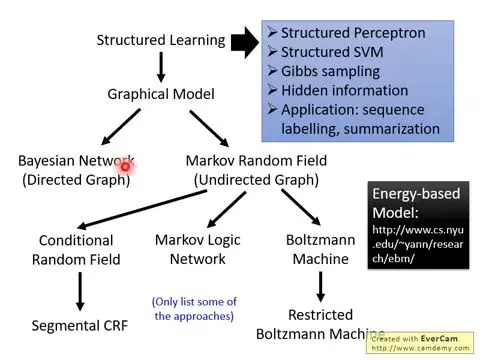
Introduction P1 - 01:24:56
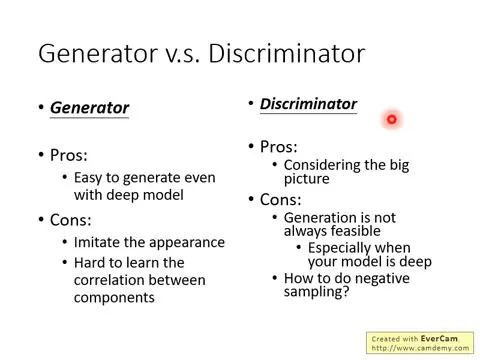
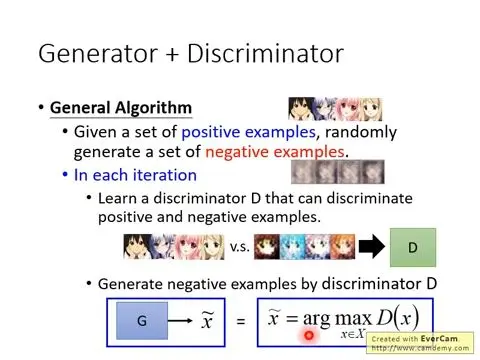
Introduction P1 - 01:27:60
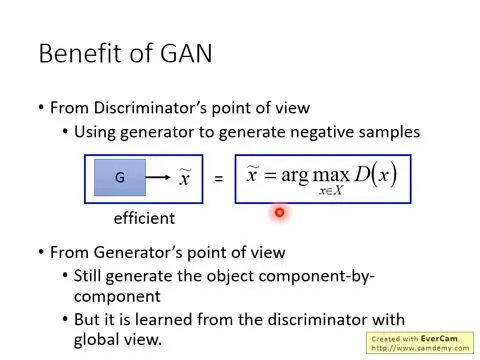