【中英双语】AI营销来了,这四种误区需要先行避开
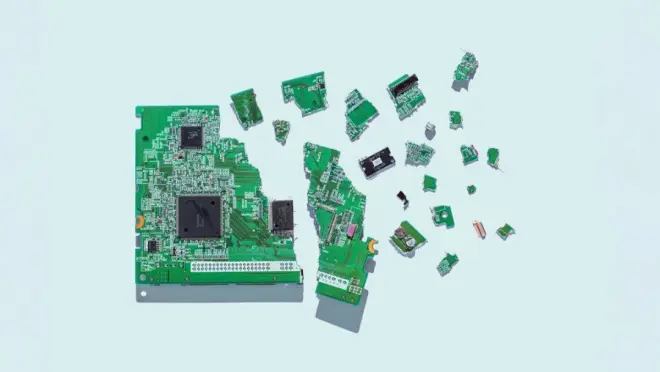
Why You Aren't Getting More from Your Marketing AI
伊娃·阿斯卡尔萨(Eva Ascarza) 迈克尔·罗斯(Michael Ross)布鲁斯·哈迪(Bruce G.S. Hardie) | 文
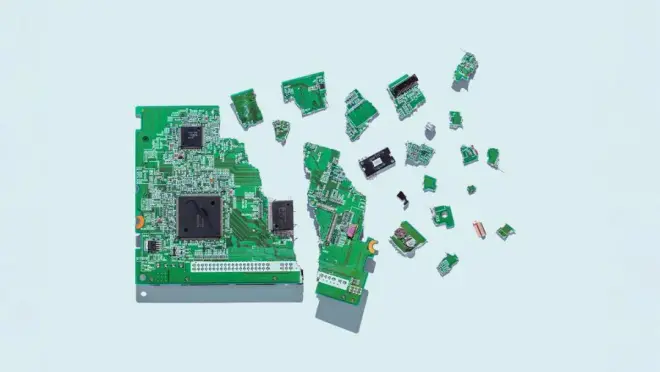
When a large telecom company’s marketers set out to reduce customer churn, they decided to use artificial intelligence to determine which customers were most likely to defect. Armed with the AI’s predictions, they bombarded the at-risk customers with promotions enticing them to stay. Yet many left despite the retention campaign. Why? The managers had made a fundamental error: They had asked the algorithm the wrong question. While the AI’s predictions were good, they didn’t address the real problem the managers were trying to solve.
大型电信企业的营销人员着手降低客户流失率,决定运用人工智能确定哪些客户会转投竞争对手。在AI预测的辅助下,他们用各种促销活动“轰炸”可能流失的顾客,想吸引这部分顾客留下,但很多顾客还是离开了。原因何在?因为他们犯了一个根本性的错误:向算法提出的问题不对。AI预测得很准,但解决的并不是营销者真正要尝试解决的问题。
That kind of scenario is all too common among companies using AI to inform business decisions. In a 2019 survey of 2,500 executivesconducted by Sloan Management Review and the Boston Consulting Group, 90% of respondents said that their companies had invested in AI, but fewer than 40% of them had seen business gains from it in the previous three years.
这种情况在利用AI辅助业务决策的公司屡见不鲜。2019年《斯隆管理评论》和波士顿咨询公司开展的一项涉及2500位高管的问卷调查中,90%的参与者表示自己的公司投资了AI,但在过去三年里看到成效的却不到40%。
In our academic, consulting, and nonexecutive director roles, we have studied and advised more than 50 companies, examining the main challenges they face as they seek to leverage AI in their marketing. This work has allowed us to identify and categorize the errors marketers most frequently make with AI.
我们在学术、咨询和非常务董事的工作中,曾对五十多家公司做过研究或提供过建议。我们考察了这些公司尝试利用AI辅助营销的过程中遇到的主要挑战。这项工作让我们总结出营销者在AI应用方面最常犯的错误。
Failure to Ask the Right Question
没能提出正确的问题
The real concern of the managers at our telecom firm should not have been identifying potential defectors; it should have been figuring out how to use marketing dollars to reduce churn. Rather than asking the AI who was most likely to leave, they should have asked who could best be persuaded to stay—in other words, which customers considering jumping ship would be most likely to respond to a promotion. Just as politicians direct their efforts at swing voters, managers should target actions toward swing customers. By giving the AI the wrong objective, the telecom marketers squandered their money on swaths of customers who were going to defect anyway and underinvested in customers they should have doubled down on.
上述电信公司的营销经理真正关心的并不是找出可能流失的顾客,而是设法利用营销资金降低流失率。与其问AI最有可能离开的是哪些顾客,不如问打算离开的顾客里哪些人最有可能被说服继续留下——换言之,考虑离开的顾客里有哪些会对促销活动做出回应。就像政客争取游离选民一样,管理者也应当瞄准尚未确定心意的游离客户。电信公司的营销团队向AI提出错误的问题,把钱浪费在无论如何都会离开的一小部分客户身上,而对原本应该双倍投入的游离客户却投资不足。
In a similar case, marketing managers at a gaming company wanted to encourage users to spend more money while they were playing its game. The marketers asked the data science team to figure out what new features would most increase users’ engagement. The team used algorithms to tease out the relationship between possible features and the amount of time customers spent playing, ultimately predicting that offering prizes and making the public ranking of users’ positions more prominent would keep people in the game longer. The company made adjustments accordingly, but new revenues didn’t follow. Why not? Because managers, again, had asked the AI the wrong question: how to increase players’ engagement rather than how to increase their in-game spending. Because most users didn’t spend money inside the game, the strategy fell flat.
还有另一个相似的例子:某家游戏公司希望鼓励用户在游戏里多花钱。营销者让数据科学团队找出最能增加用户投入度的新功能。团队用算法整理出了可以添加的新功能和玩家投入的时间之间的关系,最后做出预测:提供奖励并突出用户排名,能让玩家停留时间增加。公司于是做出相应的调整,但收入并无起色。原因何在?因为管理者又问错了问题:增加玩家投入度,并不等于增加玩家给游戏充的钱,因为大部分用户玩游戏不会花钱。这个战略失败了。
At both companies, marketing managers failed to think carefully about the business problem being addressed and the prediction needed to inform the best decision. AI would have been extremely valuable if it had predicted which telecom customers would be most persuadable and which game features would increase players’ spending.
两个公司的营销经理都没有仔细考虑需要解决的问题究竟是什么、做出最佳决策需要怎样的预测。假如用AI预测哪些用户能被挽留、哪些游戏功能最能促使玩家花钱,将会发挥极大的作用。
Failure to Recognize the Difference Between the Value of Being Right and the Costs of Being Wrong
没有分别认识到问对问题的价值和问错问题的代价
AI’s predictions should be as accurate as possible, shouldn’t they? Not necessarily. A bad forecast can be extremely expensive in some cases but less so in others; likewise, superprecise forecasts create more value in some situations than in others. Marketers—and, even more critically, the data science teams they rely on—often overlook this.
AI的预测应当尽可能地准确,不是吗?不一定。不好的预测在部分情况下可能令企业付出异常昂贵的代价,但其他时候的花费则会低一些;同样,极其准确的预测在部分情况下能够发挥更大的价值。营销者乃至其背后的数据科学团队都常常忽略这一点。
Consider the consumer goods company whose data scientists proudly announced that they’d increased the accuracy of a new sales-volume forecasting system, reducing the error rate from 25% to 17%. Unfortunately, in improving the system’s overall accuracy, they increased its precision with low-margin products while reducing its accuracy with high-margin products. Because the cost of underestimating demand for the high-margin offerings substantially outweighed the value of correctly forecasting demand for the low-margin ones, profits fell when the company implemented the new, “more accurate” system.
例如,某消费品公司的数据科学团队骄傲地宣布他们提升了新的销售量预测系统的准确度,将误差率从25%降低到17%。可惜提升了总体准确度,低利润产品相关的准确度也一并提升,高利润产品的准确度却有所下降。低估高利润产品需求的代价远远高于准确预测低利润产品需求的价值,因此这个“更准确”的新系统反而导致公司利润下降。
It’s important to recognize that AI’s predictions can be wrong in different ways. In addition to over- or underestimating results, they can give false positives (for instance, identifying customers who actually stay as probable defectors) or false negatives (identifying customers who subsequently leave as unlikely defectors). The marketer’s job is to analyze the relative cost of these types of errors, which can be very different. But this issue is often ignored by, or not even communicated to, the data science teams that build prediction models, who then assume all errors are equally important, leading to expensive mistakes.
必须认识到AI预测会出现各种不同的偏误。除了高估和低估,还有可能出现误报(比如将原本就会留下的顾客判定为可能离开)和漏报(将会离开的顾客判定为不会离开)。这几类偏误的相对成本相差很大,营销者需要对此进行分析。可是,这个问题往往被建立预测模型的数据科学团队忽略,或者根本不会告知数据团队。于是数据团队默认所有偏误都同等重要,造成昂贵的错误。
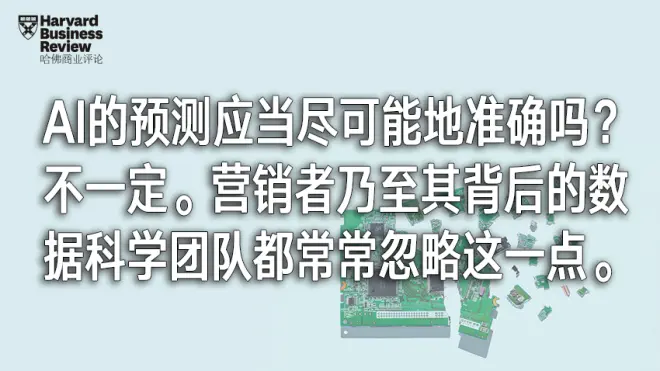
Failure to Leverage Granular Predictions
未能利用更细致的预测
Firms generate torrents of customer and operational data, which standard AI tools can use to make detailed, high-frequency predictions. But many marketers don’t exploit that capability and keep operating according to their old decision-making models. Take the hotel chain whose managers meet weekly to adjust prices at the location level despite having AI that can update demand forecasts for different room types on an hourly basis. Their decision-making process remains a relic of an antiquated booking system.
企业会产生大量的顾客数据和运营数据,标准化AI工具可以用于进行高频率的细致预测。但许多营销者并不开发这种能力,依然用旧的决策模型指导运营。例如某连锁酒店,管理者每周开会调整区域价格,却将可以每小时更新不同房型需求预测的AI闲置。他们的决策流程依然延续着过时的预订系统的做法。
Another major impediment is managers’ failure to get the granularity and frequency of their decisions right. In addition to reviewing the pace of their decision-making, they should ask whether decisions based on aggregate-level predictions should draw on more finely tuned predictions. Consider a marketing team deciding how to allocate its ad dollars on keyword searches on Google and Amazon. The data science team’s current AI can predict the lifetime value of customers acquired through those channels. However, the marketers might get a higher return on ad dollars by using more-granular predictions about customer lifetime value per keyword per channel.
另一个大的障碍是管理者未能准确理解决策的粒度和频度。在评估决策步调的时候,管理者应当思考,基于整体预测的决策是否还应当参考更加细致的预测进行调整。例如某营销团队要将广告资金分拨给谷歌和亚马逊上搜索关键词时显示的广告,数据科学团队目前的AI可以预测通过这些渠道获得的顾客的终身价值,但若能参考关于每个渠道、每个关键词的顾客终身价值的细致预测,同样的广告资金将能获得更高的回报。
Communication Breakdowns
沟通不良
In addition to constantly guarding against the types of errors we’ve described, marketing managers have to do a better job of communicating and collaborating with their data science teams—and being clear about the business problems they’re seeking to solve. That isn’t rocket science, but we often see marketing managers fall short on it.
除了提防以上误区,营销经理还应当更好地与数据科学团队交流合作,明白自己究竟要解决的是什么问题。这项工作并不复杂,但我们却时常看到营销经理在这方面有所欠缺。
Several things get in the way of productive collaboration. Some managers plunge into AI initiatives without fully understanding the technology’s capabilities and limitations. They may have unrealistic expectations and so pursue projects AI can’t deliver on, or they underestimate how much value AI could provide, so their projects lack ambition. Either situation can happen when senior managers are reluctant to reveal their lack of understanding of AI technologies.
有几个因素会妨碍高效合作。一些管理者尚未完全理解AI技术的能力和局限就匆忙行动。他们可能抱有不切实际的期望,试图实行AI无法实现的项目,抑或低估AI可以提供的价值,项目缺乏野心。高层管理者倘若不愿承认自己对AI技术理解有限,就可能会出现这两种情况中的一种。
Data science teams are also complicit in the communication breakdown. Often, data scientists gravitate toward projects with familiar prediction requirements, whether or not they are what marketing needs. Without guidance from marketers about how to provide value, data teams will often remain in their comfort zone. And while marketing managers may be reluctant to ask questions (and reveal their ignorance), data scientists often struggle to explain to nontechnical managers what they can and can’t do.
数据科学团队也有责任。数据团队往往被预测需求较熟悉的项目吸引,不顾真正的营销需求。如果没有营销者关于如何提供价值的指导,数据团队会一直停留在舒适区。营销经理可能不愿提问(因为会暴露自己的无知),而数据团队常常很难向非技术人员解释他们能做到什么、做不到什么。
Marketing needs AI. But AI needs marketing thinking to realize its full potential. This requires the marketing and data science teams to have a constant dialogue so that they can understand how to move from a theoretical solution to something that can be implemented.
营销需要AI。但AI需要营销思维,才能充分发挥潜力。这需要营销和数据科学团队保持沟通,唯其如此才能了解如何将理论解决方案应用于实践。
伊娃·阿斯卡尔萨是哈佛商学院工商管理学贾克斯基家族教席副教授。迈克尔·罗斯是为零售企业提供云数据分析的Dynamic-Action公司联合创始人、伦敦商学院执行研究员。布鲁斯·哈迪是伦敦商学院营销学教授。
蒋荟蓉 | 译 牛文静 | 校 时青靖 | 编辑
本文有删节,原文参见《哈佛商业评论》中文版2021年7月刊《AI营销潜力为何无法充分发挥》