每天一篇经济学人 | Artificial intelligence's ...
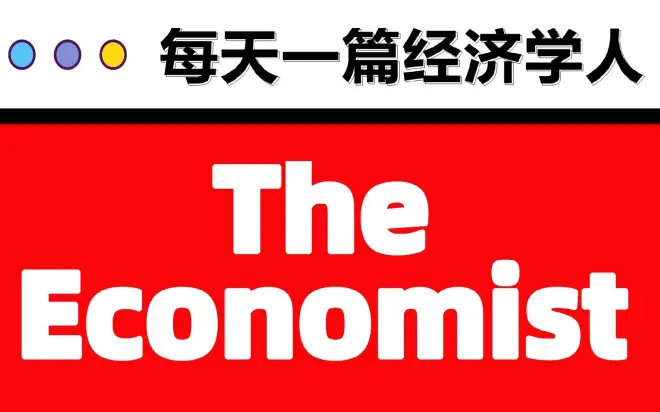
How smarter AI will change creativity
更智能的人工智能将如何改变创造力
Foundation models
基础模型(大模型)
The promise and perils of a breakthrough in machine intelligence
机器智能突破的前景和风险
Picture a computer that could finish your sentences, using a better turn of phrase; or use a snatch of melody to compose music that sounds as if you wrote it (though you never would have); or solve a problem by creating hundreds of lines of computer code—leaving you to focus on something even harder. In a sense, that computer is merely the descendant of the power looms and steam engines that hastened the Industrial Revolution. But it also belongs to a new class of machine, because it grasps the symbols in language, music and programming and uses them in ways that seem creative. A bit like a human.
【1】a turn of phrase 措词
想象一下,有这样一台电脑,它可以用更好的措辞完成你的句子;或者可以用一段旋律来作曲,就好像是你写的一样(虽然你从来没有写过);或者可以通过写数百行代码来解决一个问题,从而让你专注于更困难的事情。从某种意义上说,计算机只是加速工业革命的动力织机和蒸汽机的后代。但它也属于一类新的机器,因为它掌握语言、音乐和编程中的符号,并能以看似有创意的方式使用它们。有点像人类。
The “foundation models” that can do these things represent a breakthrough in artificial intelligence, or AI. They, too, promise a Revolution, but this one will affect the high-status brainwork that the Industrial Revolution never touched. There are no guarantees about what lies ahead—after all, AI has stumbled in the past. But it is time to look at the promise and perils of the next big thing in machine intelligence.
能够完成这些事情的“基础模型”代表着人工智能的突破。它们也承诺会带来一场革命,但这场革命将影响工业革命从未触及的高级脑力劳动。未来的情况无法保证,毕竟,人工智能在过去也曾遭遇过挫折。但是时候看看机器智能领域下一个重大事件的前景和风险了。
Foundation models are the latest twist on “deep learning” (dl), a technique that rose to prominence ten years ago and now dominates the field of AI. Loosely based on the networked structure of neurons in the human brain, dl systems are “trained” using millions or billions of examples of texts, images or sound clips. In recent years the ballooning cost, in time and money, of training ever-larger dl systems had prompted worries that the technique was reaching its limits. Some fretted about an “AI winter”. But foundation models show that building ever-larger and more complex dl does indeed continue to unlock ever more impressive new capabilities. Nobody knows where the limit lies.
基础模型是“深度学习的最新发展,该技术在10年前崭露头角,如今在人工智能领域占据主导地位。深度学习系统大致基于人类大脑神经元的网络结构,可以通过使用数百万或数十亿个文本、图像或声音片段样本进行“训练”。近年来,训练规模越来越大的深度学习系统的时间和金钱成本不断膨胀,这让人们担心这项技术正在达到极限。一些人担心“人工智能的冬天”。但基础模型显示,构建更大、更复杂的深度学习模型确实会继续“解锁”更重要的新功能。没有人知道极限在哪里。
The resulting models are a new form of creative, non-human intelligence. The systems are sophisticated enough both to possess a grasp of language and also to break the rules coherently. A dog cannot laugh at a joke in the New Yorker, but an ai can explain why it is funny—a feat that is, frankly, sometimes beyond readers of the New Yorker. When we asked one of these models to create a collage using the title of this leader and nothing more, it came up with the cover art for our American and Asian editions, pictured (we tried to distract our anxious human designers with a different cover in our European editions).
由此产生的模型是一种创造性的、非人类智能的新形式。这些系统足够复杂,既能掌握语言,又能连贯一致地打破规则。小狗不会因为《纽约客》上的笑话而发笑,但人工智能可以解释为什么它有趣,坦率地说,这一“壮举”有时超出了《纽约客》的读者的能力。当我们让其中一个模型用这篇文章的标题创作一幅拼贴画时,它创造了我们美国版和亚洲版的封面图,如图所示(我们试图在欧洲版本,使用不同封面来分散我们焦虑的人类设计师的注意力)。
Foundation models have some surprising and useful properties. The eeriest of these is their “emergent” behaviour—that is, skills (such as the ability to get a joke or match a situation and a proverb) which arise from the size and depth of the models, rather than being the result of deliberate design. Just as a rapid succession of still photographs gives the sensation of movement, so trillions of binary computational decisions fuse into a simulacrum of fluid human comprehension and creativity that, whatever the philosophers may say, looks a lot like the real thing. Even the creators of these systems are surprised at their power.
【1】eerie 怪异恐怖的
基础模型有一些令人惊讶和有用的特性。其中最可怕的是它们的“突发”行为——也就是说,技能(比如理解笑话或匹配情景和谚语的能力)是通过大规模和深层模型获得的,而不是经过深思熟虑设计的结果。就像快速连续的静态照片给人一种“移动”的感觉一样,数万亿的二元计算决策融合成一个有着易变的人类理解和创造力的模拟物,不管哲学家怎么说,这看起来很像真实的东西。即使是这些系统的创造者也对它们的威力感到惊讶。
This intelligence is broad and adaptable. True, foundation models are capable of behaving like an idiot, but then humans are, too. If you ask one who won the Nobel prize for physics in 1625, it may suggest Galileo, Bacon or Kepler, not understanding that the first prize was awarded in 1901. However, they are also adaptable in ways that earlier ais were not, perhaps because at some level there is a similarity between the rules for manipulating symbols in disciplines as different as drawing, creative writing and computer programming. This breadth means that foundation models could be used in lots of applications, from helping find new drugs using predictions about how proteins fold in three dimensions, to selecting interesting charts from datasets and dealing with open-ended questions by trawling huge databases to formulate answers that open up new areas of inquiry.
这种智能具有广泛性和适应性。确实,基础模型有可能表现得像傻瓜一样,但人类也一样。如果你问谁获得了1625年的诺贝尔物理学奖,答案可能是伽利略、培根或开普勒,他们不知道诺贝尔物理学奖是1901年颁发的。然而,它们也具有早期人工智能系统所不具备的适应性,也许是因为在某种程度上,绘画、创意写作和计算机编程等不同学科的符号操作规则之间存在相似之处。这种广度意味着基础模型可以应用于很多领域,从通过预测蛋白质在三维空间中的折叠方式以发现新药,到从数据集中选择有趣的图表,以及通过搜索庞大的数据库来制定答案,以处理开放式问题,这开辟了新查询领域。
That is exciting, and promises to bring great benefits, most of which still have to be imagined. But it also stirs up worries. Inevitably, people fear that ais creative enough to surprise their creators could become malign. In fact, foundation models are light-years from the sentient killer-robots beloved by Hollywood. Terminators tend to be focused, obsessive and blind to the broader consequences of their actions. Foundational ai, by contrast, is fuzzy. Similarly, people are anxious about the prodigious amounts of power training these models consume and the emissions they produce. However, ais are becoming more efficient, and their insights may well be essential in developing the technology that accelerates a shift to renewable energy.
这是令人兴奋的,并有望带来巨大的好处,其中大部分好处仍有待想象。但它也引发了担忧。人们不可避免地担心,人工智能系统的创造力足以让其创造者感到惊讶,这可能会变得有害。事实上,基础模型与好莱坞钟爱的有感知能力的杀人机器人相差极大。终结者倾向于专注、偏执,对自己行为的更广泛后果视而不见。相比之下,基础人工智能是模糊的。同样,人们对训练这些模型所消耗的巨大电力及其排放感到焦虑。然而,人工智能系统正变得越来越高效,它们的见解对于开发加速向可再生能源转变的技术方面很可能至关重要。
A more penetrating worry is over who controls foundation models. Training a really large system such as Google’s palm costs more than $10m a go and requires access to huge amounts of data—the more computing power and the more data the better. This raises the spectre of a technology concentrated in the hands of a small number of tech companies or governments.
一个更尖锐的担忧是关于谁控制着基础模型。训练一个像谷歌的PaLM这样的大型系统一次花费超过1000万美元,并且需要访问大量的数据——计算能力越强,数据越多越好。这引发了技术集中在少数科技公司或政府手中的担忧。
If so, the training data could further entrench the world’s biases—and in a particularly stifling and unpleasant way. Would you trust a ten-year-old whose entire sense of reality had been formed by surfing the internet? Might X- and American-trained ais be recruited to an ideological struggle to bend minds? What will happen to cultures that are poorly represented online?
如果是这样的话,训练数据可能会以一种特别令人窒息和不愉快的方式进一步加深世界的偏见。你会相信一个十岁的孩子吗? 他对现实的全部感知都是通过网上冲浪形成的。X和美国训练的人工智能系统会不会被吸纳到一场意识形态斗争中来“扭曲”人们的思想?对于那些在网络上表现不佳的文化,会发生什么?
And then there is the question of access. For the moment, the biggest models are restricted, to prevent them from being used for nefarious purposes such as generating fake news stories. Openai, a startup, has designed its model, called dall-e 2, in an attempt to stop it producing violent or pornographic images. Firms are right to fear abuse, but the more powerful these models are, the more limiting access to them creates a new elite. Self-regulation is unlikely to resolve the dilemma.
然后是访问的问题。目前,最大的模型应用受限,以防止它们被用于邪恶的目的,如制造假新闻。OpenAI是一家创业公司,它设计了一种名为DALL- E 2的模型,试图阻止它产生暴力或色情图片。公司担心模型滥用是对的,但这些模型越强大,对它们的使用以创造新的“精英”就越受限。自我监管不太可能解决这一困境。
Bring on the RevolutionFor years it has been said that ai-powered automation poses a threat to people in repetitive, routine jobs, and that artists, writers and programmers were safer. Foundation models challenge that assumption. But they also show how ai can be used as a software sidekick to enhance productivity. This machine intelligence does not resemble the human kind, but offers something entirely different. Handled well, it is more likely to complement humanity than usurp it.
多年来,有人说人工智能驱动的自动化对从事重复性、例行工作的人构成了威胁,对艺术家、作家和程序员更安全。基础模型对这种假设提出了挑战。但它们也展示了人工智能如何作为软件助手来提高生产力。这种机器智能与人类不同,但提供了一些完全不同的东西。如果处理得好,它更有可能与人类“互补”,而不是“取代”人类。