Chapter 1 Neural Networks and Deep Learning
课程视频: https://www.bilibili.com/video/BV1FT4y1E74V?p=7&vd_source=d0416378a50b5f05a80e1ed2ccc0792f
对应内容:
Chapter 1: Neural Networks and Deep Learning
Week 1: Introduction to Deep Learning
Week 2: Basics of Neural Network programming
2.1 Binary Classification
2.2 Logistic Regression
2.3 Logistic Regression Cost Function
2.4 Gradient Descent
2.5 Derivatives
2.6 More Derivative Examples
2.7 Computation Graph
2.8 Derivatives with a Computation Graph
2.9 Logistic Regression Gradient Descent
2.10 Gradient Descent on m Examples
2.11 Vectorization
2.12 More Examples of Vectorization
2.13 Vectorizing Logistic Regression
2.14 Vectorizing Logistic Regression's Gradient
2.15 Broadcasting in Python
2.16 A note on python or numpy vectors
2.17 Quick tour of Jupyter/iPython Notebooks
2.18 Explanation of logistic regression cost function
Week 3: Shallow neural networks
3.1 Neural Network Overview
3.2 Neural Network Representation
3.3 Computing a Neural Network's output
3.4 Vectorizing across multiple examples
3.5 Justification for vectorized implementation
3.6 Activation functions
3.7 why need a nonlinear activation function?
3.8 Derivatives of activation functions
3.9 Gradient descent for neural networks
3.10 Backpropagation intuition
3.11 Random+Initialization
Week 4: Deep Neural Networks
4.1 Deep L-layer neural network
4.2 Forward and backward propagation
4.3 Forward propagation in a Deep Network
4.4 Getting your matrix dimensions right
4.5 Why deep representations?
4.6 Building blocks of deep neural networks
4.7 Parameters vs Hyperparameters
4.8 What does this have to do with the brain?
笔记:
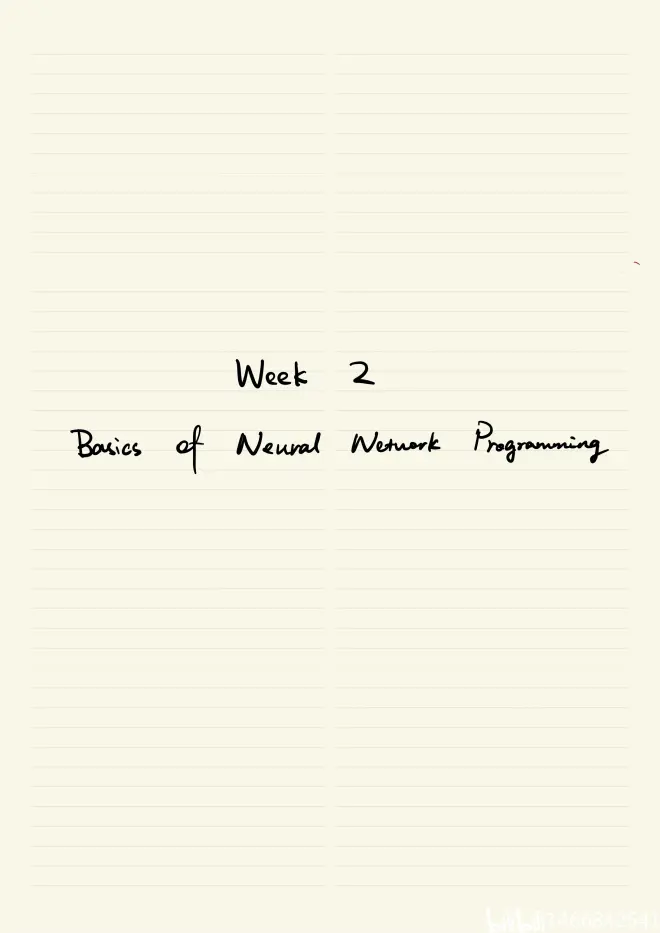
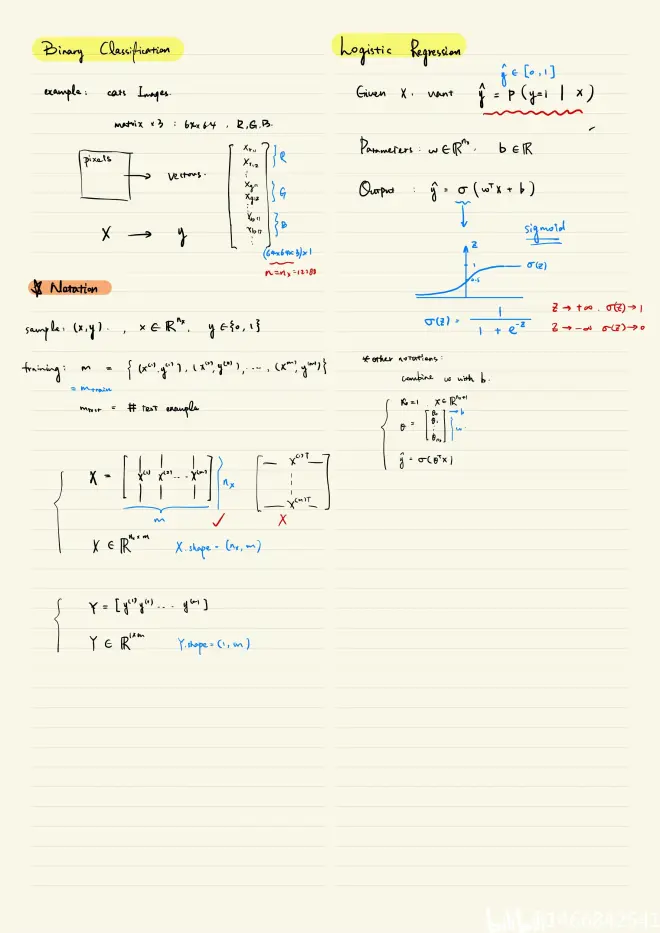
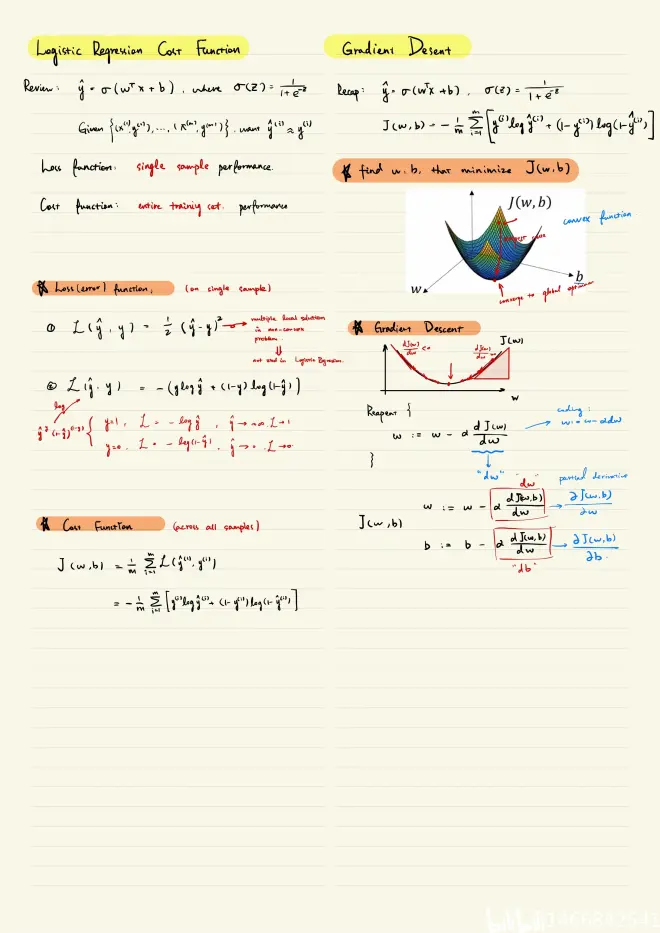
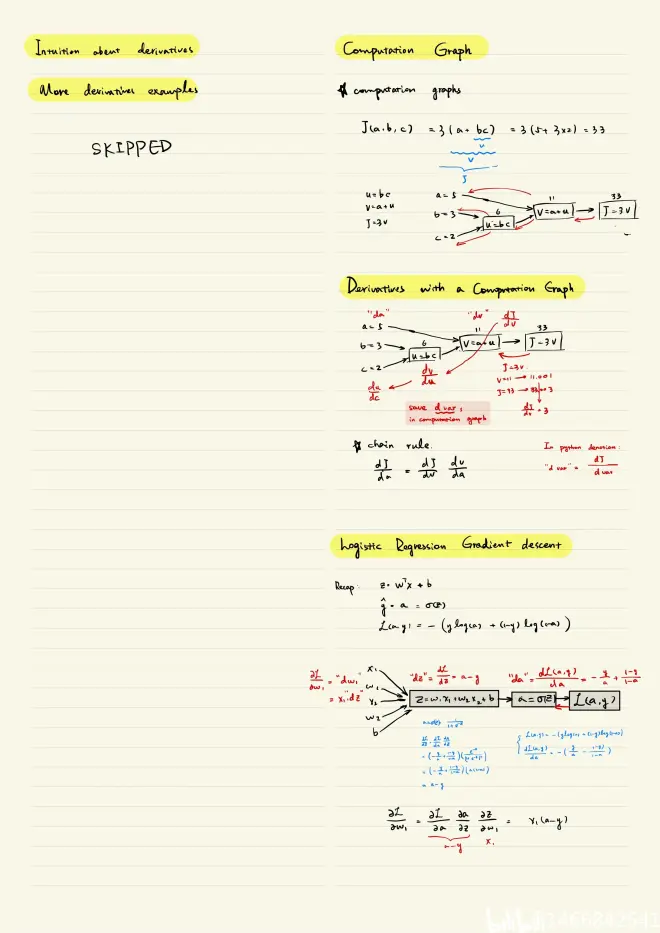
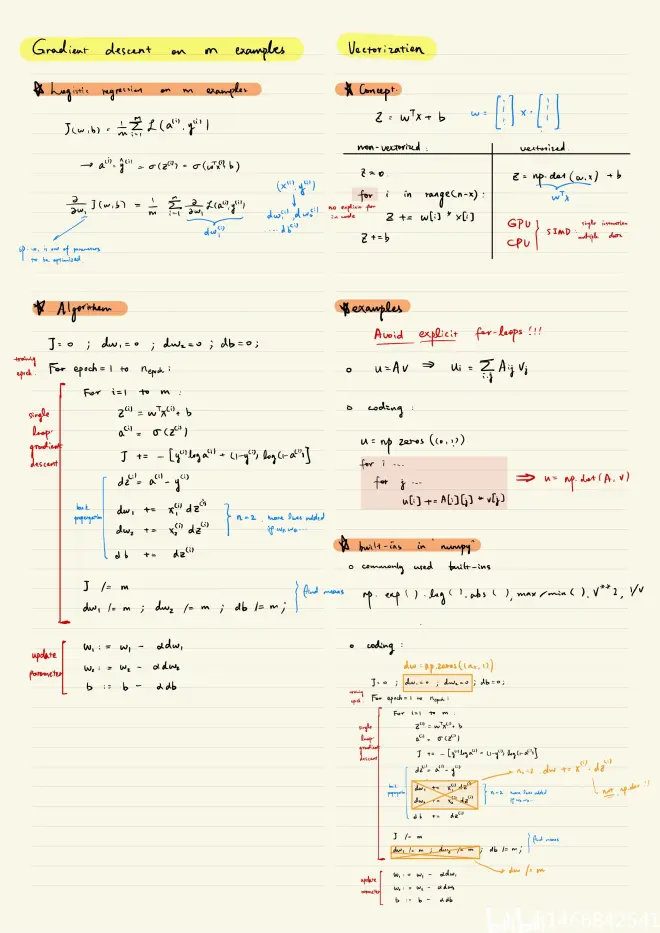
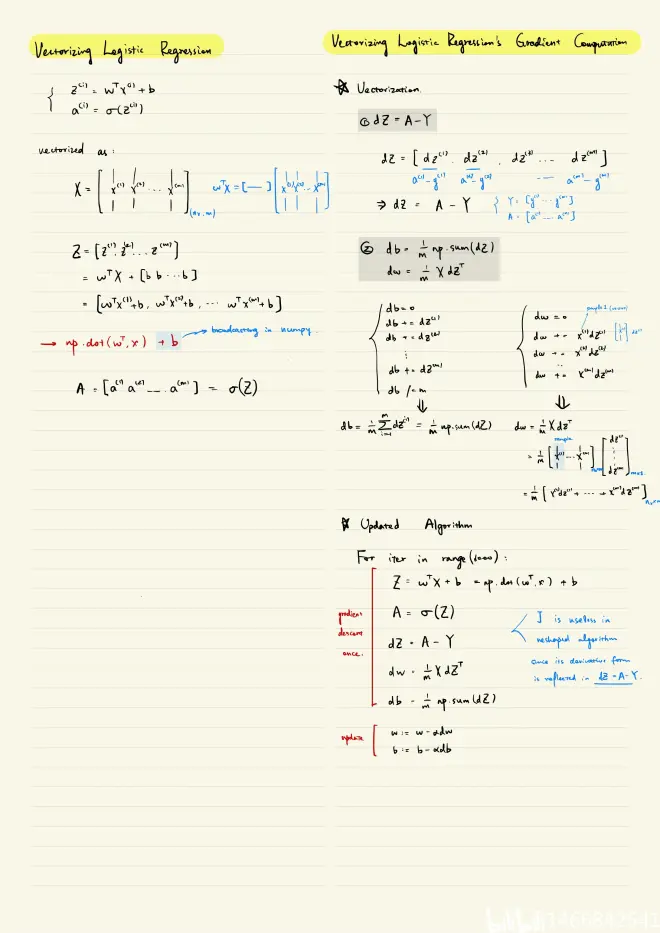
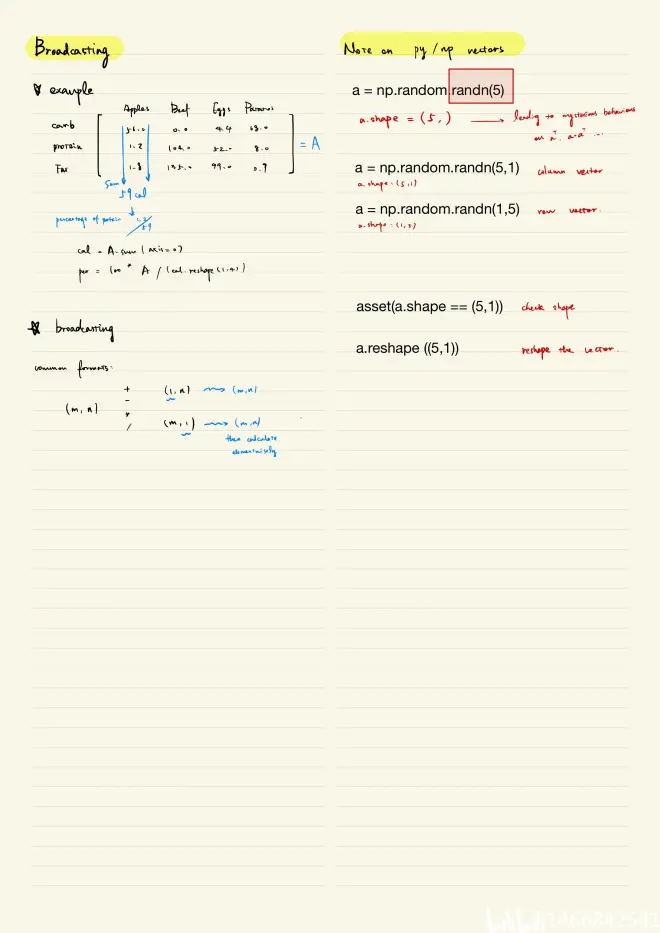
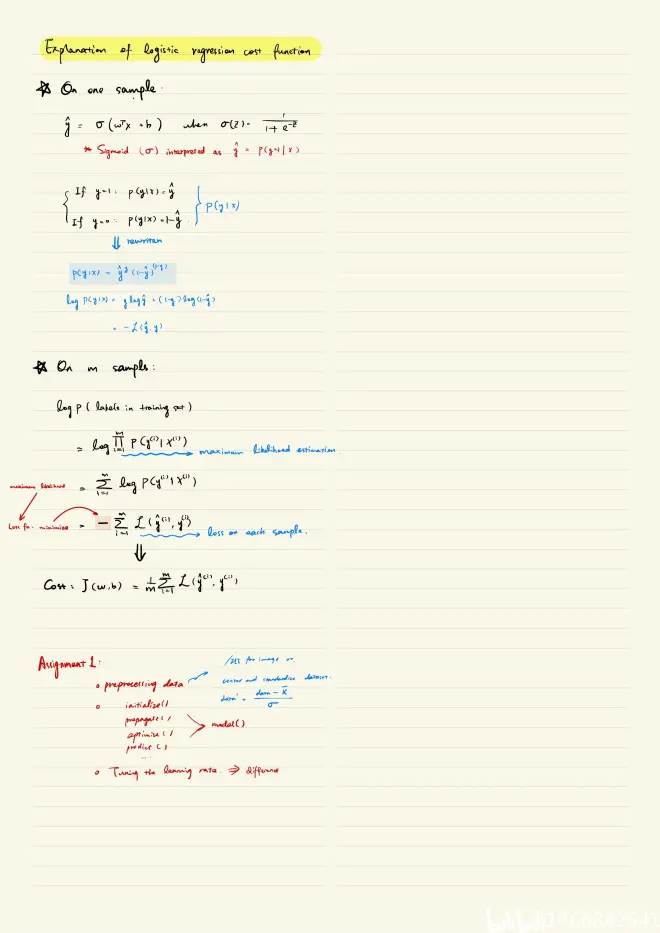
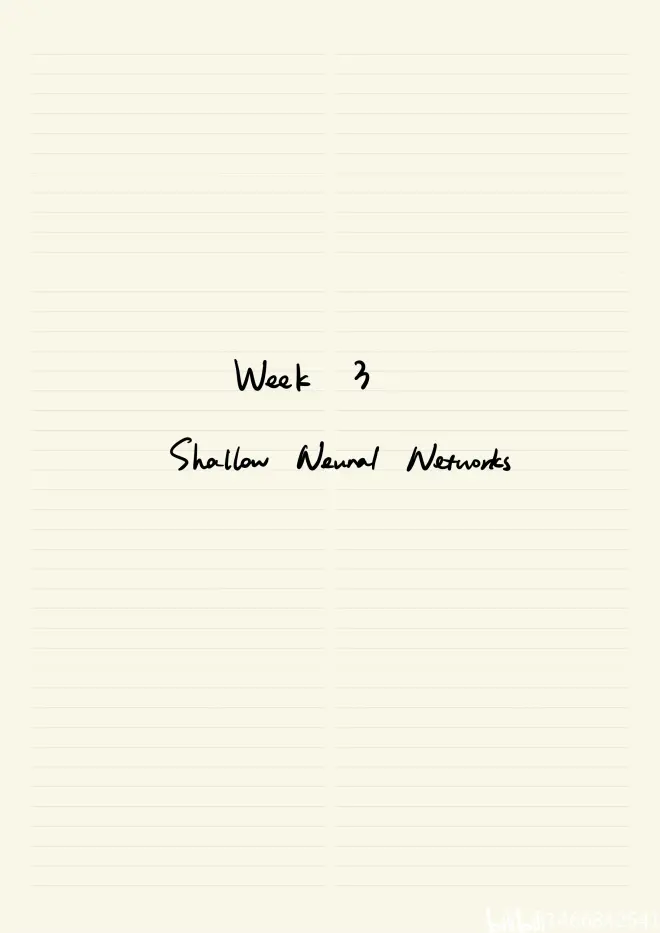
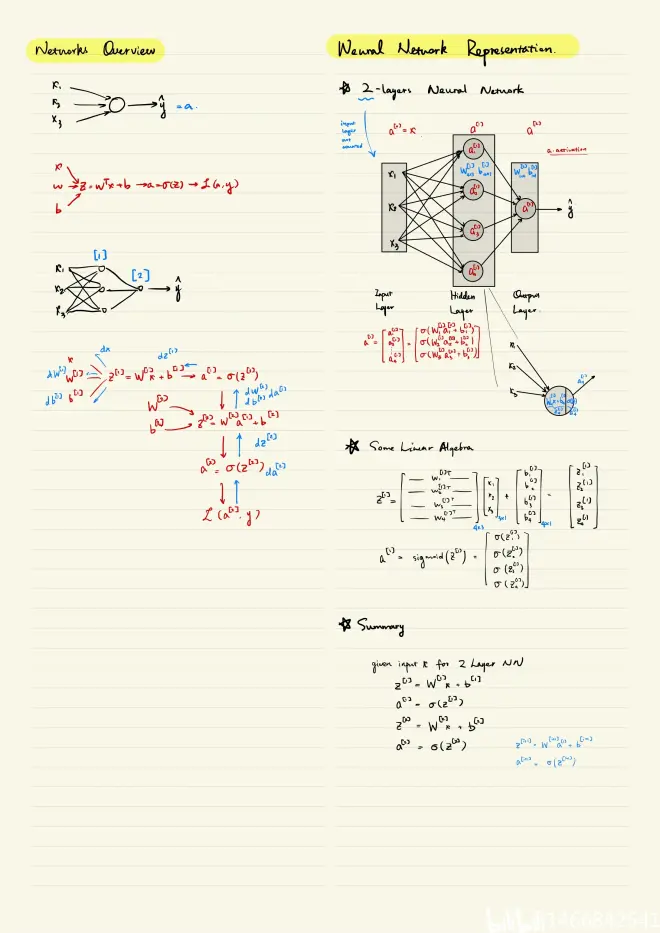
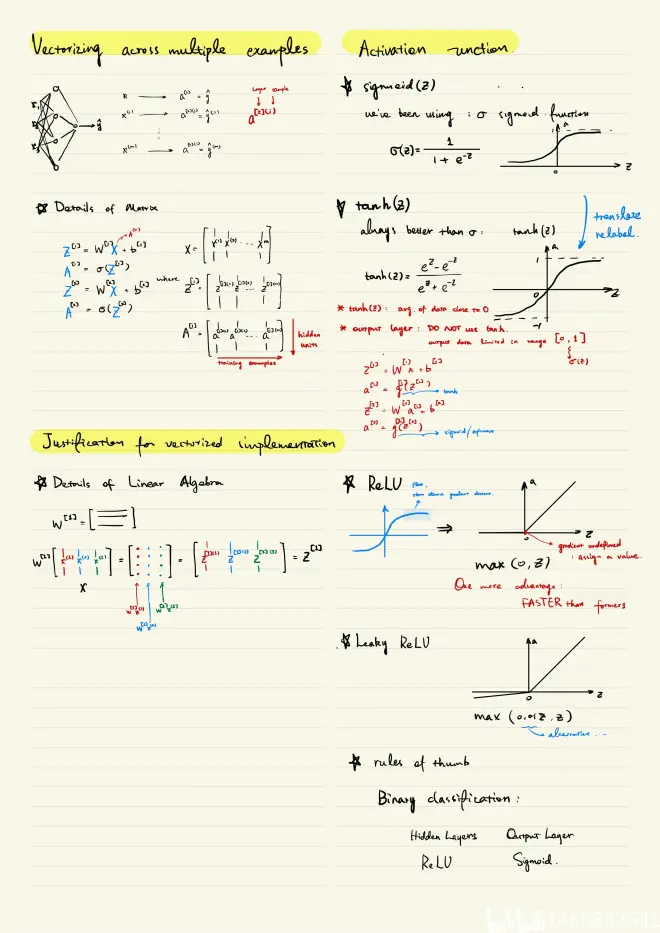
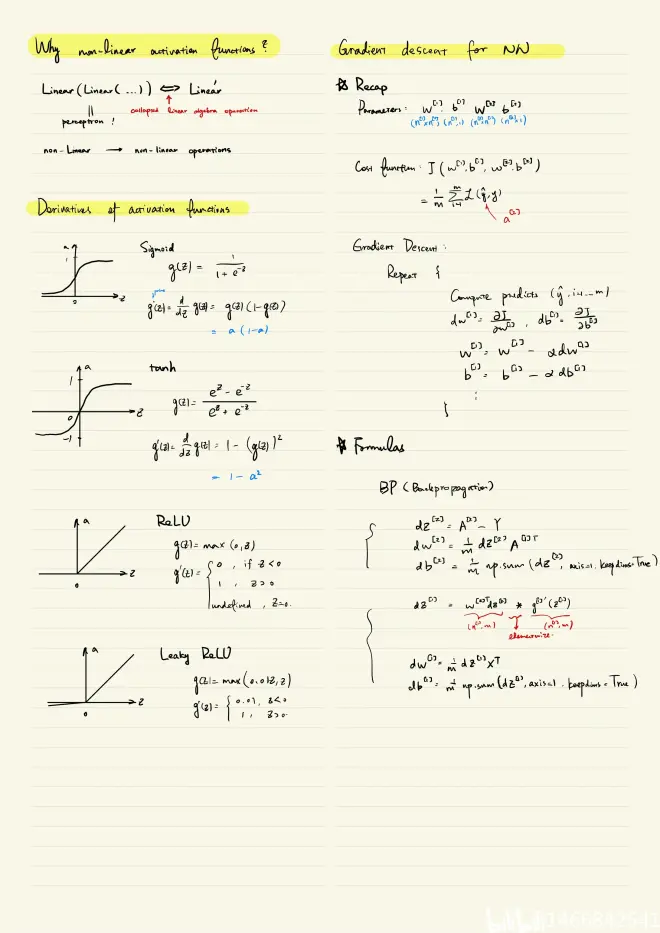
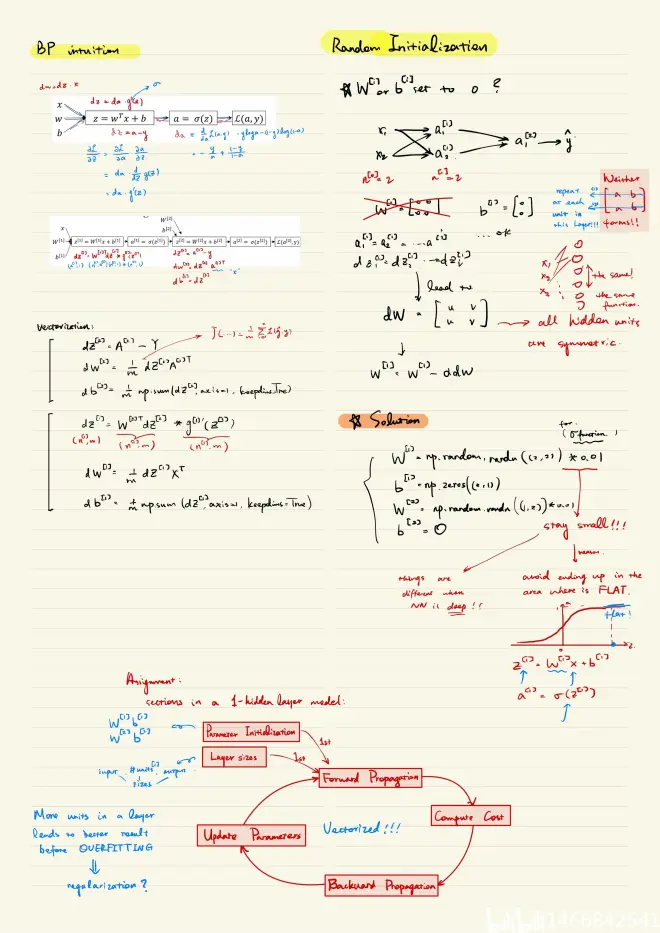
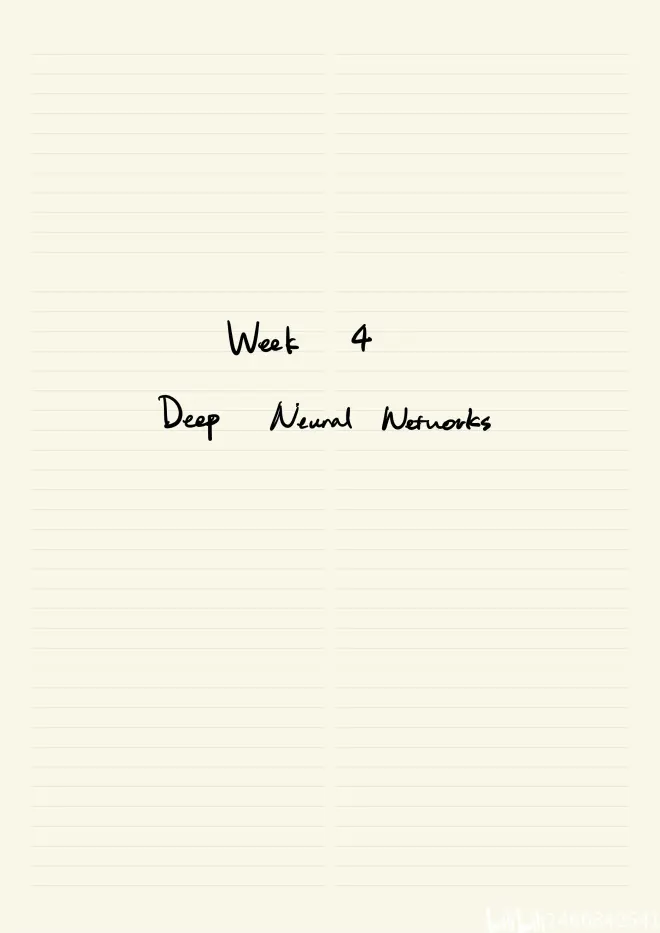
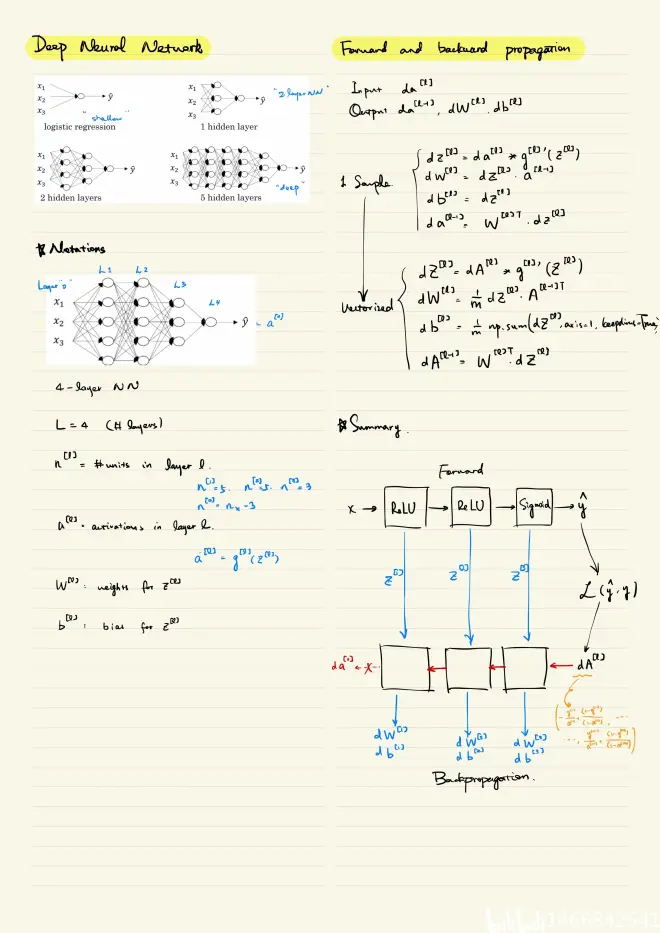
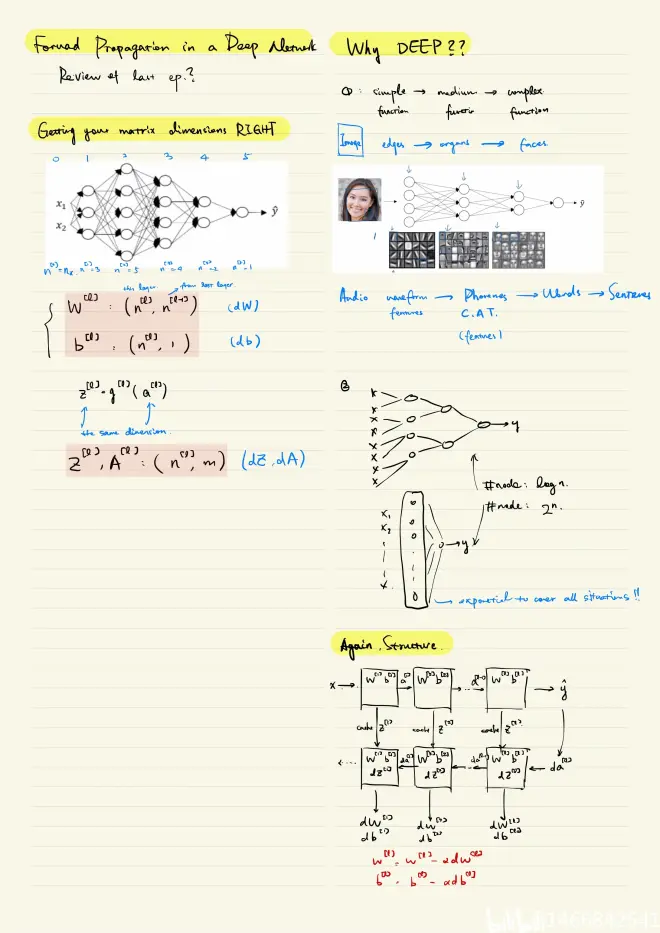
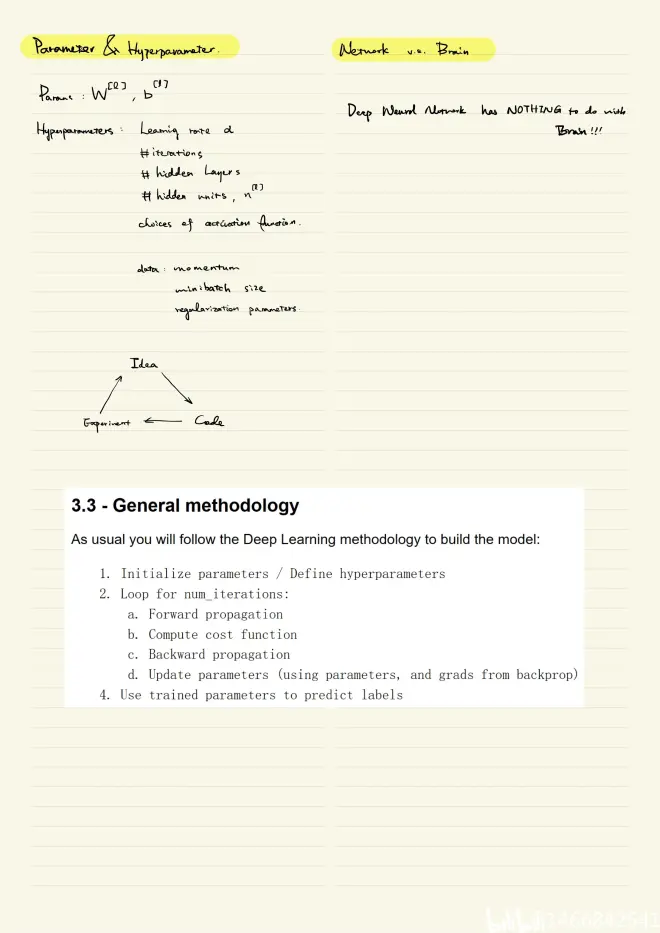